The event will be held over Microsoft Teams Live (opens in new tab) as well as in person for select talks. A lightweight registration is required. For logistical reasons, attendees will only be able to ask questions in Teams chat. Moderators will select the questions and mention your name and institution (if you include them) if your question is selected.
One-time registration provides access to all seminars in the series, making it easy to attend the sessions that work with your schedule. You’ll receive reminders with event details and a link to join in advance of each event.
The Familiarity Hypothesis: Explaining the Behavior of Deep Open Set Methods

Tom Dietterich
CoRIS Institute, Oregon State University
Date: February 2, 2023 | 2:00 PM IST (UTC+5:30)
Virtual: Microsoft Teams
-
In many applications, computer vision object recognition systems encounter objects belonging to categories unseen during training. Hence, the set of possible categories is an open set. Detecting such “novel category” objects is usually formulated as an anomaly detection problem. Anomaly detection algorithms for feature-vector data identify anomalies as outliers, but outlier detection has not worked well in deep learning. Instead, methods based on the computed logits of object recognition networks give state-of-the-art performance. This talk proposes the Familiarity Hypothesis that these methods succeed because they are detecting the absence of familiar learned features. This talk will review evidence from the literature and from our own experiments that support this hypothesis. It then experimentally tests a set of predicted consequences of this hypothesis that provide additional support. The talk will conclude with a discussion of whether familiarity detection is an inevitable consequence of representation learning. The results reveal a second fundamental assumption of statistical learning beyond the usual stationary/iid assumption—namely, that the features available to the classifier can capture variation exhibited by data points belonging to novel categories, and, more generally, by data points that lie outside the training distribution.
-
Tom Dietterich (AB Oberlin College 1977; MS University of Illinois 1979; Ph.D. Stanford University 1984) is Distinguished Professor Emeritus in the School of Electrical Engineering and Computer Science at Oregon State University. He is one of the pioneers of the field of machine learning and has authored more than 225 refereed publications and two books. His current research topics include robust artificial intelligence, robust human-AI systems, and applications in sustainability. He is the primary moderator of the cs.LG category on arXiv and was awarded the AAAI Distinguished Service Award in 2022 and the ACML Distinguished Contribution Award in 2020.
On Learning-Aware Mechanism Design
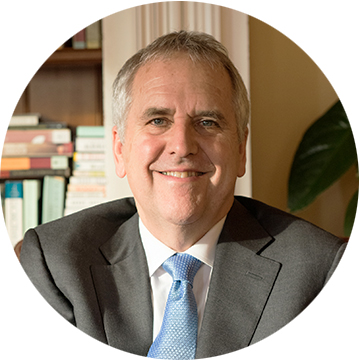
Michael I. Jordan
University of California, Berkeley
Date: January 5, 2023 | 10:00–11:30 AM IST (UTC+5:30)
Virtual: Microsoft Teams
-
Statistical decisions are often given meaning in the context of other decisions, particularly when there are scarce resources to be shared. Managing such sharing is one of the classical goals of microeconomics, and it is given new relevance in the modern setting of large, human-focused datasets, and in data-analytic contexts such as classifiers and recommendation systems. I’ll discuss several recent projects that aim to explore the interface between machine learning and microeconomics, including leader/follower dynamics in strategic classification, a Lyapunov theory for matching markets with transfers, and the use of contract theory as a way to design mechanisms that perform statistical inference.
-
Michael I. Jordan (opens in new tab) is the Pehong Chen Distinguished Professor in the Department of Electrical Engineering and Computer Science and the Department of Statistics at the University of California, Berkeley. He received his Masters in Mathematics from Arizona State University, and earned his PhD in Cognitive Science in 1985 from the University of California, San Diego. He was a professor at MIT from 1988 to 1998. His research interests bridge the computational, statistical, cognitive, biological and social sciences. Prof. Jordan is a member of the National Academy of Sciences, a member of the National Academy of Engineering, a member of the American Academy of Arts and Sciences, and a Foreign Member of the Royal Society. He is a Fellow of the American Association for the Advancement of Science. He was a Plenary Lecturer at the International Congress of Mathematicians in 2018. He received the Ulf Grenander Prize from the American Mathematical Society in 2021, the IEEE John von Neumann Medal in 2020, the IJCAI Research Excellence Award in 2016, the David E. Rumelhart Prize in 2015, and the ACM/AAAI Allen Newell Award in 2009. He gave the Inaugural IMS Grace Wahba Lecture in 2022, the IMS Neyman Lecture in 2011, and an IMS Medallion Lecture in 2004. He is a Fellow of the AAAI, ACM, ASA, CSS, IEEE, IMS, ISBA and SIAM.
In 2016, Prof. Jordan was named the «most influential computer scientist» worldwide in an article in Science, based on rankings from the Semantic Scholar search engine.
Designing AI Systems with Steerable Long-Term Dynamics
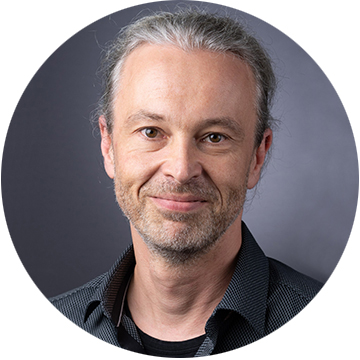
Prof. Thorsten Joachims
Cornell University
Date: November 2, 2022 | 3:00 PM IST (UTC+5:30)
Virtual: Microsoft Teams
-
The feedback that users provide through their choices (e.g. clicks, purchases) is one of the most common types of data readily available for training autonomous systems, and it is widely used in online platforms. However, naively training systems based on choice data may only improve short-term engagement, but not the long-term sustainability of the platform. In this talk, I will discuss some of the pitfalls of engagement-maximization, and explore methods that allow us to supplement engagement with additional criteria that are not limited to individual action-response metrics. The goal is to give platform operators a new set of macroscopic interventions for steering the dynamics of the platform, providing a new level of abstraction that goes beyond the engagement with individual recommendations or rankings.
-
Thorsten Joachims is a Professor in the Department of Computer Science and in the Department of Information Science at Cornell University, and he is an Amazon Scholar. His research interests center on a synthesis of theory and system building in machine learning, with applications in information access, language technology, and recommendation. His past research focused on counterfactual and causal inference, learning to rank, structured output prediction, support vector machines, text classification, learning with preferences, and learning from implicit feedback. He is an ACM Fellow, AAAI Fellow, KDD Innovations Award recipient, and member of the ACM SIGIR Academy.
-
Prof. Soumen Chakrabarti, IIT Bombay & Manish Gupta, Microsoft Research
A journey from ML and NNs to NLP and Beyond: Just more of the same isn’t enough?
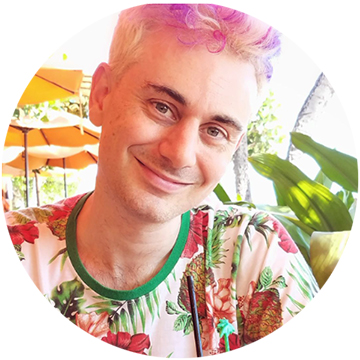
Prof. Jason Weston
Meta AI & NYU
Date: October 17, 2022 | 6:30 PM IST (UTC+5:30)
Virtual: Microsoft Teams
-
The first half of the talk will look back on the last two decades of machine learning, neural network and natural language processing research for dialogue, through my personal lens, to discuss advances that have been made and the circumstances in which they happened — to try to give clues of what we should be working on for the future. The second half will dive deeper into some current first steps in those future directions, in particular trying to fix the problems of neural generative models to enable deeper reasoning with short and long-term coherence, and to ground such dialogue agents to an environment where they can act and learn. We will argue that just scaling up current techniques, while a worthy investigation, will not be enough to solve these problems.
-
Jason Weston is a research scientist at Meta AI, USA and a Visiting Research Professor at NYU. He earned his PhD in machine learning at Royal Holloway, University of London and at AT&T Research in Red Bank, NJ (advisors: Alex Gammerman, Volodya Vovk and Vladimir Vapnik) in 2000. From 2000 to 2001, he was a researcher at Biowulf technologies. From 2002 to 2003 he was a research scientist at the Max Planck Institute for Biological Cybernetics, Tuebingen, Germany. From 2003 to 2009 he was a research staff member at NEC Labs America, Princeton. From 2009 to 2014 he was a research scientist at Google, NY. His interests lie in statistical machine learning, with a focus on reasoning, memory, perception, interaction and communication. Jason has published over 100 papers, including best paper awards at ICML and ECML, and a Test of Time Award for his work «A Unified Architecture for Natural Language Processing: Deep Neural Networks with Multitask Learning», ICML 2008 (with Ronan Collobert). He was part of the YouTube team that won a National Academy of Television Arts & Sciences Emmy Award for Technology and Engineering for Personalized Recommendation Engines for Video Discovery. He was listed as the 16th most influential machine learning scholar at AMiner and one of the top 50 authors in Computer Science in Science.
-
Prathosh A P, IISc Bangalore & Sunayana Sitarama, Microsoft Research
Deep Learning for Video Understanding
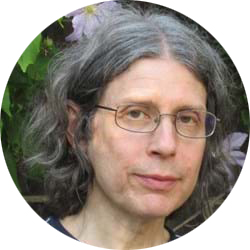
Prof. Andrew Zisserman
University of Oxford
Date: August 17, 2022 | 2:30 PM IST (UTC+5:30)
In-person: Faculty Hall, Indian Institute of Science, Bangalore
Virtual: Microsoft Teams
-
One of the long term aims of computer vision is video understanding: to be able to recognize the visual content of the video and describe what is happening. Deep learning has led to tremendous progress on many of the required visual tasks such as object recognition, human face recognition and human pose prediction.
This talk will be in three parts, and will cover progress on the tasks of human action recognition and object discovery in video. Deep networks for visual representations are very data hungry in training, and one of the key challenges is obtaining sufficient data for supervised learning.
In the first part of the talk, we describe self-supervision for deep learning where the supervision uses prediction from within the video stream, or multi-modal prediction from the audio and visual streams, in order to learn the visual representation. In the second part, we describe how self-supervision with particular network models can be used to discover objects, such as animals moving in the video, and their effects. In the final part of the talk we move onto weak supervision from text using discriminative or generative training. Once the networks are trained, a language model can be used to search for videos given a text description, or generate a text description given a video.
-
Andrew Zisserman is a Royal Society Research Professor at the Department of Engineering Science, University of Oxford, where he heads the Visual Geometry Group (VGG). His research has investigated and made contributions to computer vision, including: multiple view geometry, visual recognition, and large scale retrieval in images and video. He has authored over 500 peer reviewed papers in computer vision, and co-edited and written several books in this area. His papers have won best paper awards at international conferences, and multiple ‘test of time’ awards. His recent research focusses on audio and visual recognition. He is a fellow of the Royal Society (FRS) and the Indian National Academy of Engineering (INAE).
-
Soma Biswas, Indian Institute of Science & Akshay Nambi, Microsoft Research
GFlowNets and System 2 Deep Learning
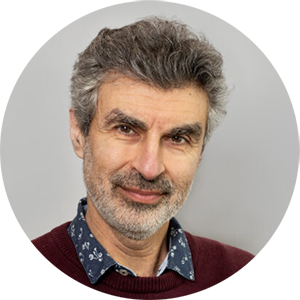
Prof. Yoshua Bengio
Université de Montréal and Mila – Quebec AI Institute
Date: June 14, 2022 | 6:30 PM—8:00 PM IST (UTC+5:30)
-
GFlowNets are instances of a larger family of approaches at the intersection of generative modeling and RL that can be used to train probabilistic inference functions in a way that is related to variational inference and opens a lot of new doors, especially for brain-inspired AI. Instead of maximizing some objective (like expected return), these approaches seek to sample latent random variables from a distribution defined by an energy function, for example a posterior distribution (given past data, current observations, etc). Recent work showed how GFlowNets can be used to sample a diversity of solutions in an active learning context. We will also discuss ongoing work to explore how to train such inference machinery for learning energy-based models, to approximately marginalize over infinitely many variables, perform efficient posterior Bayesian inference and incorporate inductive biases associated with conscious processing and reasoning in humans. These inductive biases include modular knowledge representation favoring systematic generalization, the causal nature of human thoughts, concepts, explanations and plans and the sparsity of dependencies captured by reusable relational or causal knowledge. Many open questions remain to develop these ideas, which will require many collaborating minds!
-
Recognized worldwide as one of the leading experts in artificial intelligence, Yoshua Bengio is most known for his pioneering work in deep learning, earning him the 2018 A.M. Turing Award, “the Nobel Prize of Computing,” with Geoffrey Hinton and Yann LeCun. He is a Full Professor at Université de Montréal, and the Founder and Scientific Director of Mila – Quebec AI Institute. He co-directs the CIFAR Learning in Machines & Brains program as Senior Fellow and acts as Scientific Director of IVADO.
In 2019, he was awarded the prestigious Killam Prize and in 2021, became the second most cited computer scientist in the world. He is a Fellow of both the Royal Society of London and Canada, Knight of the Legion of Honor of France and Officer of the Order of Canada. Concerned about the social impact of AI and the objective that AI benefits all, he actively contributed to the Montreal Declaration for the Responsible Development of Artificial Intelligence.
-
Where on Earth is AI Headed?
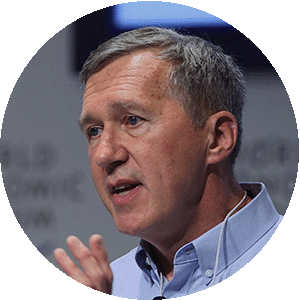
Prof. Tom M. Mitchell
Carnegie Mellon University
Date: May 10, 2022 | 4:00 PM IST (UTC+5:30)
-
Ten years ago computers could not understand spoken words well, but today we routinely speak to our mobile phones, and computer vision algorithms have now reached human or super-human level performance for many types of images. Self-driving cars are already appearing on our streets, and a deep network called GPT-3 writes surprisingly human-like paragraphs of text. What is coming next? This talk will look forward to suggest what technical progress we might work toward and even expect over the coming years, and its possible impacts on our lifestyles, products, business models, society and international politics.
-
Tom M. Mitchell is the Founders University Professor in the School of Computer Science at Carnegie Mellon University, where he founded the world’s first academic Machine Learning Department. Mitchell’s research explores machine learning theory, algorithms and applications, as well as the impact of AI on society. He has testified to the U.S. Congress several times on AI impacts on society, and he co-chaired the 2017 U.S. National Academy study on “Information Technology, Automation, and the U.S. Workforce.” Mitchell advises a variety of young and old companies internationally on their AI product and business strategies, and his research has been featured in popular press from the New York Times, to CCTV (China’s national television network), to CBS’s 60 Minutes. He is an elected member of the U.S. National Academy of Engineering, the American Academy of Arts and Sciences, and a Fellow and Past President of the Association for the Advancement of Artificial Intelligence (AAAI).
-
Learning to Walk
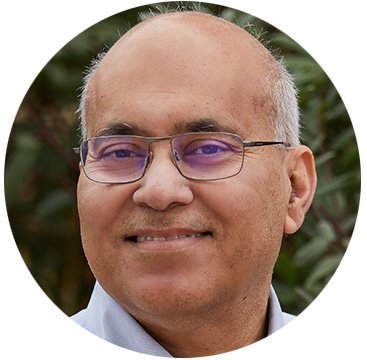
Prof. Jitendra Malik
University of California, Berkeley
Date: March 04, 2022 | 09:00 AM IST (UTC+5:30)
-
Legged locomotion is commonly studied and programmed as a discrete set of structured gait patterns, like walk, trot, gallop. However, studies of children learning to walk (Adolph et al) show that real-world locomotion is often quite unstructured and more like “bouts of intermittent steps”. We have developed a general approach to walking which is built on learning on varied terrains in simulation and then fast online adaptation (fractions of a second) in the real world. This is made possible by our Rapid Motor Adaptation (RMA) algorithm. RMA consists of two components: a base policy and an adaptation module, both of which can be trained in simulation. We thus learn walking policies that are much more flexible and adaptable. In our set-up gaits emerge as a consequence of minimizing energy consumption at different target speeds, consistent with various animal motor studies. We then incrementally add a navigation layer to the robot from onboard cameras and tightly couple it with locomotion via proprioception without retraining the walking policy. This is enabled by the use of additional safety monitors which are trained in simulation to predict the safe walking speed for the robot under varying conditions and also detect collisions which might get missed by the onboard cameras. The planner then uses these to plan a path for the robot in a locomotion aware way. You can see our robot walking at https://www.youtube.com/watch?v=nBy1piJrq1A (opens in new tab).
-
Jitendra Malik is Arthur J. Chick Professor of EECS at UC Berkeley. He obtained his B.Tech degree in EE from IIT Kanpur in 1980 and a PhD in Computer Science from Stanford University in 1985. His research has spanned computer vision, machine learning, modeling of human vision, computer graphics, and most recently robotics. He has advised more than 70 PhD students and postdocs, many of whom are now prominent researchers. His honors include numerous best paper prizes, the 2013 Distinguished Researcher award in computer vision, the 2016 ACM/AAAI Allen Newell Award, the 2018 IJCAI Award for Research Excellence in AI, and the 2019 IEEE Computer Society’s Computer Pioneer Award for “leading role in developing Computer Vision into a thriving discipline through pioneering research, leadership, and mentorship”. He is a member of the US National Academy of Sciences, the US National Academy of Engineering, and the American Academy of Arts and Sciences.
-