
Peter Lee
微软全球资深副总裁
美国计算机协会(ACM)院士
-
Dr. Peter Lee is Corporate Vice President, AI & Research, at Microsoft. He is responsible for incubating research projects that lead to new products and services. Examples of past and current projects include: deep neural networks (opens in new tab) for computer vision and the simultaneous language translation feature in Skype (opens in new tab); new silicon (opens in new tab) and post-silicon (opens in new tab) computer architectures for Microsoft’s cloud (opens in new tab); experimental under-sea datacenters (opens in new tab); augmented-reality experiences (opens in new tab) for HoloLens and VR devices; digital storage in DNA (opens in new tab); social chatbots XiaoIce (opens in new tab) and Tay (opens in new tab); and healthcare innovation (opens in new tab). Previously, he was an Office Director at DARPA, where he led efforts that created operational capabilities in advanced machine learning, crowdsourcing, and big-data analytics, such as the DARPA Network Challenge (opens in new tab) and Nexus 7 (opens in new tab). He was formerly the Head of Carnegie Mellon University’s computer science department (opens in new tab). A thought leader in technological innovation, Dr. Lee served on President’s Commission on Enhancing National Cybersecurity (opens in new tab), led a study for the National Academies on the impact of federal research investments on economic growth (opens in new tab), and testified before the US House Science and Technology Committee (opens in new tab) and the US Senate Commerce Committee (opens in new tab). He is widely quoted on industry trends and innovation in the New York Times (opens in new tab), MIT Technology Review (opens in new tab), Wired (opens in new tab), Fast Company (opens in new tab), The Economist (opens in new tab), ArsTechnica (opens in new tab), CNN (opens in new tab), several books (opens in new tab), and more.
-
Artisanal AI
Scientists and technologists are pursuing the dream of artificial intelligence like never before. The progress of research is clearly accelerating, and this is leading more and more people, including business leaders and scholars, to become optimistic about the prospects for practical applications of AI. At Microsoft, AI is being infused into almost every product and service, providing tremendous benefits to users. However, creating and deploying such applications of AI require a great deal of specialized expertise and hand-crafted solutions. In this sense, one might say that we are in an era of “artisanal AI.” In this presentation, I will explain more about the artisanal nature of today’s AI applications, and lay out prospects for a more industrialized future.

John Hopcroft
康奈尔大学计算机系教授,1986年图灵奖获得者
电气电子工程师学会(IEEE)及美国计算机协会(ACM)院士
-
John E. Hopcroft is the IBM Professor of Engineering and Applied Mathematics in Computer Science at Cornell University. His research centers on theoretical aspects of computer science. He was dean of Cornell’s College of Engineering from 1994 to 2001.
In 1992 he was appointed by President George H.W. Bush to the National Science Board, which oversees the National Science Foundation, and served through May 1998. He serves on Microsoft’s Technical Advisory Board for Research Asia, and the advisory boards of IIIT Delhi and Seattle University’s College of Engineering.
He is a member of the National Academy of Engineering (1989) and National Academy of Sciences (2009), and a fellow of the American Academy of Arts and Sciences, American Association for the Advancement of Science, Institute of Electrical and Electronics Engineers (IEEE), Association of Computing Machinery (ACM), and Society of Industrial and Applied Mathematics.
He has received the A.M. Turing Award (1986), IEEE Harry Goode Memorial Award (2005), Computing Research Association’s Distinguished Service Award (2007), ACM Karl V. Karlstrom Outstanding Educator Award (2009), IEEE John von Neumann Medal (2010), and China’s Friendship Medal (2016), China’s highest recognition for a foreigner. In addition, the Chinese Academy of Sciences has designated him an Einstein professor.
He has honorary degrees from Seattle University, the National College of Ireland, the University of Sydney, St. Petersburg State University in Russia, Beijing University of Technology, and Hong Kong University of Science and Technology, and is an honorary professor of the Beijing Institute of Technology, Shanghai Jiao Tong University, Chongqing University, Yunnan University, and Peking University.
He received his BS (1961) from Seattle University and his MS (1962) and PhD (1964) in electrical engineering from Stanford University.
-
The AI Revolution
There is an information revolution taking place driven by artificial intelligence. The revolution started with the support vector machine model 15 or 20 years ago but has recently been driven by deep learning. Deep learning has had tremendous success in many application areas but little is known as to why it works so effectively.
This talk will review the basics of machine learning and then present some interesting research directions in deep learning.

Lise Getoor
加州大学圣克鲁兹分校计算机科学系教授
美国人工智能学会(AAAI)院士
-
Lise Getoor is a professor in the Computer Science Department at the University of California, Santa Cruz. Her research areas include machine learning, data integration and reasoning under uncertainty, with an emphasis on graph and network data. She has over 200 publications and extensive experience with machine learning and probabilistic modeling methods for graph and network data. She is a Fellow of the Association for Artificial Intelligence, an elected board member of the International Machine Learning Society, serves on the board of the Computing Research Association (CRA), and was co-chair for ICML 2011. She is a recipient of an NSF Career Award and eleven best paper and best student paper awards. In 2014, she was recognized by KDD Nuggets as one of the emerging research leaders in data mining and data science based on citation and impact. She received her PhD from Stanford University in 2001, her MS from UC Berkeley, and her BS from UC Santa Barbara, and was a professor in the Computer Science Department at the University of Maryland, College Park from 2001-2013.
-
Big Graph Data Science: Making Useful Inferences from Graph Data
Graph data (e.g., communication data, financial transaction networks, data describing biological systems, collaboration networks, organization hierarchies, social media, etc.) is ubiquitous. While this observational data is useful, it is usually noisy, often only partially observed, and only hints at the actual underlying social, scientific or technological structures that gave rise to the interactions. One of the challenges in big data analytics lies in being able to reason collectively this kind of extremely large, heterogeneous, incomplete, and noisy interlinked data.
In this talk, I will describe some common inference patterns needed for graph data including: collective classification (predicting missing labels for nodes), link prediction (predicting edges), and entity resolution (determining when two nodes refer to the same underlying entity). I will describe some key capabilities required to solve these problems, and finally I will describe probabilistic soft logic (PSL), a highly scalable open-source probabilistic programming language being developed within my group to solve these challenges.

Raymond Mooney
德克萨斯大学奥斯汀分校计算机科学系教授兼人工智能实验室主任
美国计算机协会(ACM)及美国人工智能学会(AAAI)院士
-
Raymond J. Mooney is a Professor in the Department of Computer Science at the University of Texas at Austin. He received his Ph.D. in 1988 from the University of Illinois at Urbana/Champaign. He is an author of over 160 published research papers, primarily in the areas of machine learning and natural language processing. He was the President of the International Machine Learning Society from 2008-2011, program co-chair for AAAI 2006, general chair for HLT-EMNLP 2005, and co-chair for ICML 1990. He is a Fellow of the American Association for Artificial Intelligence, the Association for Computing Machinery, and the Association for Computational Linguistics and the recipient of best paper awards from AAAI-96, KDD-04, ICML-05 and ACL-07.
-
The Deep Learning Revolution: Progress, Promise, and Profligate Promotion
New machine learning methods for «deep» neural networks have demonstrated remarkable performance on a number of challenging AI problems and led to a recent «revolution» in AI research.
I will briefly review the history of machine learning, the basics of deep learning (including convolutional and recurrent networks), and their recent successful applications to problems in computer vision, speech and natural language processing, and game playing. I will also discuss the unfortunate «hype» that has accompanied this progress and the limitations of current methods and the overall deep learning paradigm. The goal is to present a balanced high-level picture of the current state of machine learning.
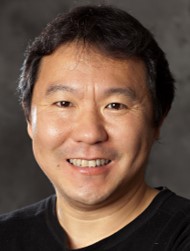
滕尚华
南加州大学计算机科学与数学系教授
美国计算机协会(ACM)院士
-
Shang-Hua Teng is currently a University Professor and Seely Mudd Professor of Computer Science and Mathematics at the University of Southern California (USC), where he was the chair of the Computer Science Department from 2009-2012. He received his Ph.D. of Computer Science from Carnegie Mellon University. Before USC, he taught at BU, UIUC, University of Minnesota, and MIT.Teng has twice won the prestigious Gödel Prize in theoretical computer science, first in 2008, for developing the theory of smoothed analysis, and then in 2015, for designing the groundbreaking nearly-linear time Laplacian solver for network systems. Both are joint work with Dan Spielman of Yale — his long-time collaborator. Citing him as, «one of the most original theoretical computer scientists in the world», the Simons Foundation named Teng a 2014 Simons Investigator, for pursuing long-term curiosity-driven fundamental research. He and his collaborators also received the best paper award at ACM Symposium on Theory of Computing (STOC) for what’s considered to be the «first improvement in 10 years» of a fundamental optimization problem — the computation of maximum flows and minimum cuts in a network. In addition, he is known for his joint work with Xi Chen and Xiaotie Deng that characterized the complexity for computing an approximate Nash equilibrium in game theory, and his joint papers on market equilibria in computational economics. He and his collaborators also pioneered the development of well-shaped Delaunay meshing algorithms for arbitrary three-dimensional geometric domains, which settled a long-term open problem in numerical simulation, also a fundamental problem in computer graphics. Software based on this development was used at the University of Illinois for the simulation of advanced rockets. Teng is also interested in mathematical board games. With his former Ph.D. student Kyle Burke, he designed and analyzed a game called Atropos, which is played on the Sperner’s triangle and based on the beautiful, celebrated Sperner’s Lemma. In 2000 at UIUC, Teng was named on the List of Teachers Ranked as Excellent by Their Students for his class, «Network Security and Cryptography». He has worked and consulted for Microsoft Research, Akamai, IBM Almaden Research Center, Intel Corporation, Xerox PARC, and NASA Ames Research Center, for which he received fifteen patents for his work on compiler optimization, Internet technology, and social networks.
Teng’s recent research interests include algorithmic theory for Big Data and network science, spectral graph theory, social-choice-theoretical approaches to community identification, and game-theoretical frameworks for understanding the interplay between influence processes and social networks. With a four-year old daughter, he has also become intensely interested in the learning theory for early childhood bilingual acquisition.
-
Scalable Algorithms for Big Data and Network Analysis
In the age of Big Data, efficient algorithms are in higher demand now more than ever before. While Big Data takes us into the asymptotic world envisioned by our pioneers, the explosive growth of problem size has also significantly challenged the classical notion of efficient algorithms: Algorithms that used to be considered efficient, according to polynomial-time characterization, may no longer be adequate for solving today’s problems. It is not just desirable, but essential, that efficient algorithms should be scalable. In other words, their complexity should be nearly linear or sub-linear with respect to the problem size. Thus, scalability, not just polynomial-time computability, should be elevated as the central complexity notion for characterizing efficient computation.
In this talk, I will discuss a family of algorithmic techniques for the design of provably-good scalable algorithms, focusing on the emerging Laplacian Paradigm, which has led to breakthroughs in scalable algorithms for several fundamental problems in network analysis, machine learning, and scientific computing. These techniques include local network exploration, advanced sampling, sparsification, and graph partitioning. Network analysis subject include four recent applications: (1) Sampling from Graphic Models (2) Network Centrality Approximation (3) Social-Influence Maximization (4) Random-Walk Sparsification.
Solutions to these problems exemplify the fusion of combinatorial, numerical, and statistical thinking in network analysis.
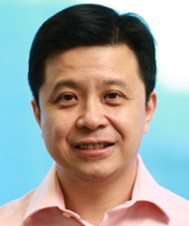
洪小文
微软全球资深副总裁,微软亚太研发集团主席兼微软亚洲研究院院长
微软杰出首席科学家,电气电子工程师学会(IEEE)院士
-
Dr. Hsiao-Wuen Hon is corporate vice president of Microsoft, chairman of Microsoft’s Asia-Pacific R&D Group, and managing director of Microsoft Research Asia. He drives Microsoft’s strategy for research and development activities in the Asia-Pacific region, as well as collaborations with academia.
Dr. Hon has been with Microsoft since 1995. He joined Microsoft Research Asia in 2004 as deputy managing director, stepping into the role of managing director in 2007. He founded and managed Microsoft Search Technology Center from 2005 to 2007 and led development of Microsoft’s search products (Bing) in Asia-Pacific. In 2014, Dr. Hon was appointed as chairman of Microsoft Asia-Pacific R&D Group.
Prior to joining Microsoft Research Asia, Dr. Hon was the founding member and architect of the Natural Interactive Services Division at Microsoft Corporation. Besides overseeing architectural and technical aspects of the award-winning Microsoft Speech Server product, Natural User Interface Platform and Microsoft Assistance Platform, he was also responsible for managing and delivering statistical learning technologies and advanced search. Dr. Hon joined Microsoft Research as a senior researcher in 1995 and has been a key contributor to Microsoft’s SAPI and speech engine technologies. He previously worked at Apple, where he led research and development for Apple’s Chinese Dictation Kit.
An IEEE Fellow and a distinguished scientist of Microsoft, Dr. Hon is an internationally recognized expert in speech technology. Dr. Hon has published more than 100 technical papers in international journals and at conferences. He co-authored a book, Spoken Language Processing, which is a graduate-level textbook and reference book in the area of speech technology used in universities around the world. Dr. Hon holds three dozen patents in several technical areas.
Dr. Hon received a Ph.D. in Computer Science from Carnegie Mellon University and a B.S. in Electrical Engineering from National Taiwan University.
-
Learning to Learn: Exploring Approaches to Help Machine and People Learn
In recent years, there have been much progress in machine learning in the areas of computer vision, speech, natural language processing, and other domains. Yet, there remain many challenging situations where better machine learning algorithms are necessary. There are cases where teaching signals and evaluation metrics are very clear. There are also scenarios where evaluation metrics can be subjective and one would need to rely on real world feedback for better learning. In this talk, I will present some of the recent work in helping machines learn from Microsoft Research Asia, such as dual learning and self-generated data learning. Furthermore, I will highlight some important challenges for machine learning. Lastly, as artificial intelligence makes bigger impact on society, people also need to adapt to enhance their skills. I will talk about some recent work on using machine to help people learn.