Presented by Peter Lee at Microsoft Research Forum, January 2024
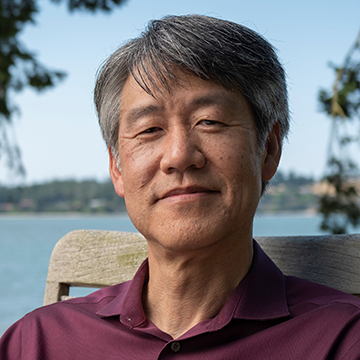
“As we work together as a research community in computer science, we are in this incredibly exciting stage, a stage of being disrupted personally as researchers … and a whole new vista of possibilities in front of us. And we are just incredibly excited within Microsoft Research to be living through this.”
– Peter Lee, CVP, Microsoft Research & Incubations
- Microsoft research copilot experience Summarize the main three points of Peter's talk
Transcript
Peter Lee, Corporate Vice President, Microsoft Research and Incubations
Peter Lee discusses how recent developments in AI have transformed the way Microsoft approaches research.
Microsoft Research Forum, January 30, 2024
PETER LEE: Hi. I’m really pleased and excited to be here for this first Microsoft Research Forum, a series that we have here out of Microsoft Research to carry out some important conversations with the research and scientific community.
This past year has been quite a memorable one. Just some incredible advances, particularly in AI, and I’ll spend a little bit of time talking about AI here to get us started. But before doing that, I thought I would try to at least share how I see what is happening in the broader context of scientific disruption. And to do that, I want to go all the way back to the 1700s and the emerging science of biology, the science of living things. Actually, in the 1700s, it was well understood by the end of that century that all living things were made up of cells—everything from trees and plants to bugs, animals, and human beings. But a fundamental scientific mystery that lingered for decades was, where do cells come from?
And a prevailing theory of that was this concept of cell crystallization. It has been understood in other areas that sometimes hard materials would crystallize into existence from fluid materials. And so the thought was that out of living fluids, under just the right conditions, cells would crystallize into existence. And a lot of biological research of the time was centered around that theory. And in fact, quite a few important and useful things came out of that line of research, research that even has impact medically today. Now of course, there was an alternative theory, which I think is credited to Robert Remak, that in fact cells get created through a process of cell division. And we know that this is true today. But it was really considered an alternative theory until Rudolf Virchow was actually able to witness the mitosis of cells, the division of cells, and in fact coined the aphorism that all cells come from other living cells. And this had a very significant impact on Virchow’s research and his research into what is now known as pathology.
Overnight, whole research legacies were rendered largely invalid because the whole concept of cell crystallization was then known to be invalid. But even the very foundational infrastructure of research at the time changed. In fact, after Virchow, to call yourself a researcher in biology, you had to have access to a new piece of research infrastructure called the microscope, and you had to be good at using it. And so while the researchers themselves of the time were not invalidated, they were disrupted in a really fundamental way. And of course, the discovery of mitosis really set biology research on the path ultimately to the discovery of DNA and the remarkable kinds of medical and biological advances we see in the field.
Now I tell that story because when I think about that story—and I learned it first from the great biology researcher and medical scientist Sid Mukherjee at Columbia—I think about what we as computer scientists are going through today. We’ve now witnessed the incredible potential power of machine learning systems at scale and of specific architectures like neural transformers. And there are many possibilities, there are many challenges, and there are many mysteries. And furthermore, the infrastructure of what we do as computer science researchers, particularly in areas related to artificial intelligence, has changed in the same way that biology researchers need access to new infrastructure like microscopes. At least that was the case in the mid-1800s, when Virchow made his discovery.
Today, for a large segment of the kinds of research that we do, we now realize we need new types of infrastructure, infrastructure such as large datasets, access to large scale GPU compute, and even other training pipelines and foundations. And what we’re seeing is that this is affecting virtually everything that we do today. And so as we work together as a research community in computer science, we are in this incredibly exciting stage, a stage of being disrupted personally as researchers—many of us as researchers finding large parts of what we had been working on being changed, disrupted, or even invalidated—and a whole new vista of possibilities in front of us. And we are just incredibly excited within Microsoft Research to be living through this. There are difficult moments, to be sure, but also a sense of joy, a joy that comes from the realization that we are now living through something that is very special and very rare.
So now what has this meant? And to do a little bit of a discussion about that, if you will permit me, I’d like to focus a little bit on the research, particularly in AI within Microsoft Research in this past year. We had the opportunity to do something unusual. And while I use the word opportunity, it was also a challenge. In our ongoing collaboration with OpenAI, when the new model that we now call GPT-4 was being made available for investigation and study within Microsoft Research—and this was toward the end of 2022—we, for various reasons, were required to do something that is exceptionally unusual for Microsoft Research, and that is to work in secret for a period of several weeks.
And this is exceptionally unusual for Microsoft Research because almost all of the research we do at Microsoft is done in collaboration with external researchers, particularly at great universities all around the world. And so really for the first time, we were doing some core research in secret, and that remained secret until the release publicly of GPT-4 in March of 2023. That March of 2023 marked the time when we were allowed finally to speak publicly about GPT-4 in the wake of OpenAI’s public announcement of that model and allowed the publication of our initial findings on our own internal study and investigation of this model. And that has led to a paper that was tantalizingly titled “Sparks of Artificial General Intelligence,” or what is now oftentimes referred to as the Sparks paper.
That Sparks paper was really a turning point for many of us in the research community. It tried to show a series of example interactions with this new large language model that defied complete explanation in terms of the emergence of apparent cognitive capabilities. It was also a somewhat edgy or even controversial paper because of our then lack of ability to fully explain the core mechanisms about where these apparent capabilities were coming from. At the same time, it was a real chance to finally reach out and establish collaborations with many of you here today, applications and collaborations to understand, to what extent are these models able to do planning? What is the nature of causal reasoning and causal inference? Counterfactual reasoning? What is the interchange between fundamental reasoning abilities of these models versus world knowledge? To what extent are [they] commonsense reasoning? Decision making in controversial or morally charged circumstances? And fundamentally, what could this mean more broadly for us as people, for the communities we live in, and for societies?
The Sparks paper was just the beginning, and with many of you, we’ve had a series of important research advances that have been deepening our understanding in these and many other areas. And we’ve been trying to put these things into action as we have also worked with our own Microsoft product developers as well as researchers and product developers in other organizations, in other companies. We’ve really had to come to grips with the impact of AI technology on the world and on people. In our internal collaborations with our Bing product team, we devoted considerable effort in trying to understand the guardrails around responsible AI. And in fact, today at Microsoft, our responsible AI organization within Microsoft has hundreds of people really forming around understanding the impact not just of the potential harms and risks that AI technologies can bring when deployed widely at scale but the broader opportunities both for benefit as well as risks on society as a whole.
So I’d like to say just a few more words about this whole concept of responsible AI. And in fact, I prefer to think of this as the area of AI and its impact on society or AI and society, for short. For us, in this new AI era, we started in a difficult space, because we devoted some of our best expertise across Microsoft but specifically Microsoft Research to building the guardrails and understanding the risks of our first integrations of GPT-4 into our products like Bing. And that devotion, in secret, ended up being noticeable to the research community after the release of ChatGPT, when we were in a somewhat difficult position in Microsoft Research of having to remain silent while the research community was starting to really delve into the research questions around AI safety of ChatGPT, in that case, and then later in GPT-4. What has happened since then, I think, is now a renaissance in our understanding, jointly with all of you, about the nature of AI risks and the whole debate around the future of AI and society—not only the future of work, which we care about a great deal in our own research here at Microsoft, but the impact on communities, on relationships, and societies in core areas like medicine and health care, in education, in finance, in law, and you name it. I’m extremely excited about what this has meant for our own research within Microsoft in an area that we call sociotechnical systems and specifically in something we call FATE: Fairness, Accountability, Transparency, and Ethics.
There has never been a more exciting time and never been larger and more challenging research questions as well as bigger and more relevant opportunities for the impact of this research. And we can’t be more excited to be working with all of you on many of these things. Within Microsoft, this has also had a transformative effect. We have evolved from having a single organization for responsible AI to now deeply integrating responsible AI and, more broadly, AI and societal impact thinking into literally every engineering group across the company as well as areas in finance, security, and our legal departments. And so as we think about the future of AI and society, we just really look forward and we will be depending on our collaborations with all of you.
Now it doesn’t just stop there, though. There are tremendous other areas. The actual costs of training, the necessity or not of scale, and the emergence of alternative models has become extremely important. And so another thread of research that has been exceptionally important in AI for us over the past year has been in the emerging area of open source and small language model development. And we’ve been very proud to have shared with the research community in open-source form a series of models called Phi. The Phi models are exceptionally interesting, because they’ve taken a new approach to the construction of training data to synthesize data to really focus on specific reasoning and problem-solving strategies as opposed to world knowledge. And this has led to a series of models starting with Phi-1 (opens in new tab), 1.5 (opens in new tab), and now Phi-2 (opens in new tab) that have been devoted to open source in the hopes of encouraging and supporting the open-research community in developing greater understanding of the transparency and the limits of these types of models, to understand better the alignment issues, and to have further explication in the pretraining phase of areas related to AI safety and risk.
We’ve also been looking at platform and model orchestration. What will this world look like in the future, where there may be many models together? And so we’ve been extremely proud of our work on AutoGen (opens in new tab). AutoGen has provided, again in the open-source community, a way to very easily and rapidly get multiple AI systems collaborating together as independent agents to solve problems more easily—to, for example, have one model interact with a human being to solve problems, another model look over their shoulders to ensure that nothing goes wrong, and maybe even a third agent, which is a human being in the loop, doing various kinds of checks and balances.
We’ve been studying tremendously about how we can extend our ability to train these models for specific domains. Our work on Orca and Orca 2 (opens in new tab) has really helped shed more light on the nature of training data. And our work on the LLaVA (opens in new tab) model specialized to medical image generation in LLaVA-Med (opens in new tab) has shown real promise for a future of specialized models devoted to aspects of medicine.
Now while I’m talking about model specialization, this interplay between specialization versus generalization has been another major theme for Microsoft Research AI over the past year. The basic question is, do we need intense specialization? And nowhere has that question been more pertinent than the area of health care and medicine. Do we need to take AI models to med school in order for them to be useful in the medical domain? The question is still a mystery to us, and in fact, we released a series of prompting scripts that would automate the creation of chain-of-thought augmentation of prompts called promptbase and its application to medicine called Medprompt that shows that GPT-4 when suitably prompted still outperforms any existing specialist model. And so to date, we still have a mystery as to the role of specialization. And furthermore, we have some hints that specialization may lead to the loss of some cognitive function, and a really fun paper to read out of Microsoft Research and our Azure division is entitled “[Who’s] Harry Potter?” where we show that even a small amount of specialized training of large language models can get a large language model to forget everything it ever knew about Harry Potter. A humorous title, but it makes an important point in deepening understanding of the role of specialization.
All of these put together, of course, is just the tip of a very, very large iceberg, one that is growing at tremendous speed. And in fact, today we are seeing so much of AI research happening in the world, in social media, at the speed of conversation, to the point where even our top-tier AI researchers feel, at times, that their heads are spinning. But working together, providing openness, providing greater access, we definitely—looking back over this past year—can see that we’ve made tremendous, tremendous progress. And it’s not just the new discoveries that we jointly have made together but also in deepening understanding about how to care and mitigate against the downstream harms and risks of AI as we see them emerge as well as the broader societal impacts about what this will mean for the future of work, for the future of life, and the future of our relationships with technology.
Now as we think more about AI, it has just infected—and I’m using that word for a reason—almost all of the research that we do across Microsoft Research, whether it’s in security and privacy, whether it’s in sociotechnical systems, whether it’s in program analysis and verification. You name it, generative AI has been having an impact. I can’t tell you how surprised and tickled I was the first time I saw our program analysis research group using GPT-4 to help synthesize loop invariants for the purposes of furthering problem verification analysis. Just really cool. A little bit amusing; maybe even a little bit scary. But just amazing no matter how you look at it.
Now when we take all that, I use the word infected because, of course, one area that has had a special place within Microsoft Research over the last few years has been in areas related to health care and medicine. And in fact, we saw early on the potential impact that GPT-4 would have in health care and medicine, and in fact, I myself coauthored—with Carey Goldberg, who is a science writer, and Zak Kohane, from Harvard Medical School—a book on the potential impact of GPT-4 on health care and medicine (opens in new tab). And we had already in place a Microsoft Research lab called Health Futures that had been working on large language models such as BioGPT (opens in new tab) and PubMedBERT (opens in new tab) for the purposes of creating knowledge bases and supporting decisions in clinical settings, and much of that in collaboration with our partners at Nuance, the makers of really the most widely used medical transcription and note-taking technologies today.
GPT-4 and the emergence of large language models have really changed everything and in fact have broadened beyond the narrow confines of health care and medicine to other parts of science, other natural sciences—the discovery of new materials, the discovery of catalysts to help climate science, the potential to take these sorts of models and make them multi-scale so that we can now start modeling weather patterns and predict weather events ahead of time.
And in recognition of all of that, we’ve created a new Microsoft Research lab called AI4Science, and we’re very proud that already we’re seeing some tremendous results from this. Working with collaborators at the Pacific Northwest National Laboratories, we very rapidly were able to synthesize the discovery of new electrolyte substances, combining sodium and lithium in ways that could be the foundation of a new generation of solid-state batteries that make a dramatically lowered use of what is oftentimes referred to as “white gold,” or lithium. And we’ve furthermore been able to work with the Global Health Drug Discovery [Institute], or GHDDI, in very rapidly discovering new inhibitors that may form the foundation of new drug treatments for diseases such as tuberculosis and coronaviruses. And so the future is just incredibly bright, and as we extend beyond language and medical images and other types of two-dimensional images to 3D-structure learning and the ability to make predictions about the structure of physical systems like molecules, we see just an incredibly bright future ahead of all of us.
As I think about our future as fellow researchers, as scientists, I just see a tremendous reason to be optimistic. You know, we’re in an era where what we do has never mattered more than it matters right now. The things that we’re working on have tremendous technological power to really empower and reach every person on the planet and make their lives better in so many different ways. And it’s something that we can just do together and go directly from the laboratory into the real world.
And so I really am hoping and I’m looking forward to us continuing in this spirit of collaboration, in the spirit of openness, to help ensure that that future is as vibrant and bright as we know it can be while at the same time being clear-eyed about the potential risks, risks that we don’t even understand or realize yet today. But together we can do what scientists have always done in the past, which is to ensure that we get as many of the benefits out of emerging new technologies while mitigating the downstream harms and risks. If we do that together, if we do it with the right spirit and attitude, I think the future is incredibly bright. I know I’m really looking forward to doing it with you.
Thank you again for joining us, and I hope you enjoy this first Microsoft Research Forum.
Related resources
- Project AutoGen
- Publication Textbooks Are All You Need