Presented by Shuxin Zheng at Microsoft Research Forum, January 2024
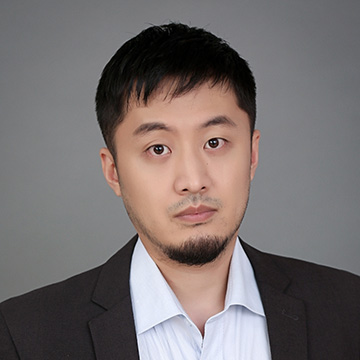
“Understanding equilibrium distributions in molecular science is challenging but exciting. … By learning about the different states and the behavior of molecules, scientists can make breakthroughs in developing new drugs, creating advanced materials, and understanding biological processes.”
– Shuxin Zheng, Principal Researcher
-
Microsoft research copilot experience Summarize the main three points of Shuxin's talk
Transcript
Shuxin Zheng, Principal Researcher, Microsoft Research AI4Science
Shuxin Zheng presents how his team uses generative AI to solve a long-standing challenge in structural biology and molecular science—predicting equilibrium distribution for molecular systems.
Microsoft Research Forum, January 30, 2024
SHUXIN ZHENG: Hi, everyone. I’m Shuxin from Microsoft Research AI4Science. Thank you for joining this exciting discussion of our latest research, called Distributional Graphormer, which uses generative AI to solve a long-standing challenge in structural biology: the prediction of equilibrium distribution.
We begin by acknowledging the groundbreaking work in protein structure prediction. However, proteins are dynamic, constantly changing their conformation. This is where our research takes a pioneering step, focusing on the equilibrium distributions of these structures versus a static image.
Understanding equilibrium distributions in molecular science is challenging but exciting because it opens up new possibilities in diverse fields. By learning about the different states and the behavior of molecules, scientists can make breakthroughs in developing new drugs, creating advanced materials, and understanding biological processes.
Our new approach, the Distributional Graphormer, brings generative AI technologies into thermodynamics, offering efficiency and accuracy to obtain the equilibrium distribution for any molecular system, far beyond traditional methods like molecular dynamics simulation. It begins with any descriptor of a molecular system. For example, the sequence of amino acids revolutionized the prediction of molecular systems’ equilibrium distribution.
Let’s dive into practical implications. Consider the case of B-Raf kinase, a protein linked to cancer. Traditional methods fail to capture its active and inactive states comprehensively. DiG, on the other hand, accurately samples these states, demonstrating its power in understanding the important dynamics.
Let’s see a real-world application. The ability of DiG to predict a range of conformations of the main proteins of SARS-CoV-2 virus provides insight that could revolutionize how we understand the viral mutations and the development of drugs. DiG can also reveal the interaction between protein and ligands and predict the binding of free energy to aid in modern drug discovery. The transition pathway of conformation can be easily obtained with DiG by a fast interpolation in latent space.
Beyond protein systems, DiG can also predict equilibrium distribution for other molecular systems. For example, this figure shows DiG predicts the density of catalyst-adsorbate systems compared with the results of DFT calculations.
In closing, DiG is a paradigm shift in molecular science—from the structure prediction and the molecular simulation to equilibrium distribution prediction with generative AI. Its potential applications are vast, touching upon areas from bioinformatics to material discovery. I invite you to explore our new findings on the arXiv paper (opens in new tab) and engage with our interactive demo (opens in new tab) to witness the future of molecular science.
Thank you for your time.