Audio-based Toxic Language Classification using Self-attentive Convolutional Neural Network
- Midia Yousefi ,
- Dimitra Emmanouilidou
2021 29th European Signal Processing Conference (EUSIPCO) |
Published by IEEE | Organized by European Association for Signal Processing (EURASIP)
The monumental increase in online social interaction activities such as social networking or online gaming is often riddled by hostile or aggressive behavior that can lead to unsolicited manifestations of cyberbullying or harassment. In this work, we develop an audio-based toxic language classifier using self-attentive Convolutional Neural Networks (CNNs). As definitions of hostility or toxicity can vary depending on the platform or application, in this work we take a more general approach for identifying toxic utterances, one that does not depend on individual lexicon terms, but rather considers the entire acoustical context of the short verse or utterance. In the proposed architecture, the self-attention mechanism captures the temporal dependency of the verbal content by summarizing all the relevant information from different regions of the utterance. The proposed audio-based self-attentive CNN model is evaluated on a public and an internal dataset and achieves 75% accuracy, 79% precision, and 80% recall in identifying toxic speech recordings.
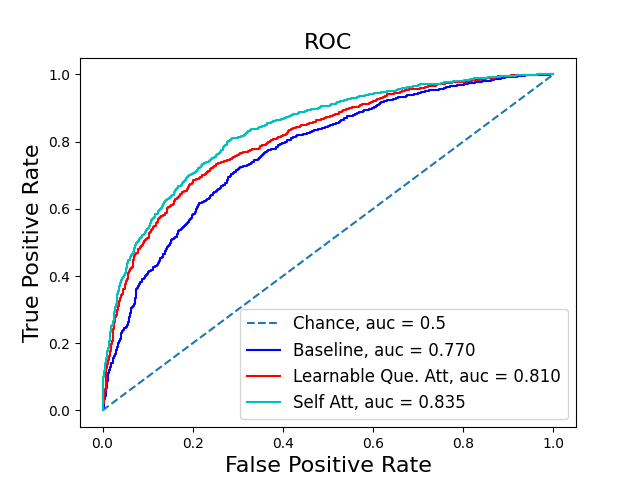
ROC curve for internal toxicity dataset, corpus A.

Accuracy plots for corpus IEMOCAP showing benefits of data augmentation.
Audio-based Toxic Language Detection
Online gaming has been growing increasingly popular recently. This highly competitive online social platform can sometimes lead to undesired behavior and create an unfriendly community for many players. The detecting of profanity and bullying have been previously explored in text-based platforms (ex. social media, Twitter/Facebook) but when it comes to speech-based applications including online gaming, the field is relatively unexplored. In this project we focus on audio-based toxic language detection, which can be a great asset in scenarios where text transcriptions are not readily available. Additionally, audio-based queues such as speech tone or pitch variation could potentially provide supplementary or orthogonal information to transcribed content and word-based features. We have developed a Self-Attentive Convolutional Neural Network architecture to carry out the detection of toxic segments…