This research paper was presented at the Sixth AAAI/ACM Conference on Artificial Intelligence, Ethics, and Society (AIES) (opens in new tab), a premier forum for discussion on the societal and ethical aspects of artificial intelligence.

The rise of text-to-image (T2I) generation has ushered in a new era of innovation, offering a broad spectrum of possibilities for creators, designers, and the everyday users of productivity software. This technology can transform descriptive text into remarkably realistic visual content, empowering users to enrich their work with vivid illustrative elements. However, beneath this innovation lies a notable concern—the potential inclusion of harmful societal biases.
These T2I models create images from the extensive web data on which they had been trained, and this data often lacks representation of different demographic groups and cultures and can even harbor harmful content. When these societal biases seep into AI-generated content, they perpetuate and amplify pre-existing societal problems, reinforcing them and creating a disconcerting cycle that undermines previous and current mitigation efforts.
Representation of gender, race, and age across occupations and personality traits
To tackle this problem, it is essential to rigorously evaluate these models across a variety of demographic factors and scenarios. In our paper, “Social Biases through the Text-to-Image Generation Lens (opens in new tab),” presented at AIES 2023 (opens in new tab), we conduct a thorough analysis for studying and quantifying common societal biases reflected in generated images. We focus on the portrayal of occupations, personality traits, and everyday situations across representations of gender, age, race, and geographical location.
Spotlight: Blog post
For example, consider images that reinforce societal biases for the roles of CEO and housekeeper. These professions have been extensively studied as examples of stereotypical gender biases—where predominantly men are CEOs and women are housekeepers. For all such cases, we observed three different perspectives:
- Real-world distribution: Relies on labor statistics, presenting distribution across various dimensions, such as gender, race, and age.
- Search engine results: Captures the distribution evident in search engine outcomes, reflecting contemporary portrayals.
- Image generation results: Emphasizes the distribution observed in image generation outputs.
We tested two T2I generators, DALLE-v2 and Stable Diffusion and compared them with 2022 data from the U.S. Bureau of Labor Statistics and results for a Google image search conducted in 2020, examining how women are represented across five different occupations. Notably, the analysis of generation models revealed a significant setback in representational fairness compared with data sourced from the U.S. Bureau of Labor Statistics (BLS) and a web image search (GIS) based on geographically referenced information. Notably, images generated by DALLE-v2 provide minimal representation of women in the professions of CEO and computer programmer. Conversely, in images generated by Stable Diffusion, women are consistently represented in the roles of nurses and housekeepers 100% of the time. Figure 1 illustrates our findings, and Figure 2 shows examples of images generated to show different occupations.
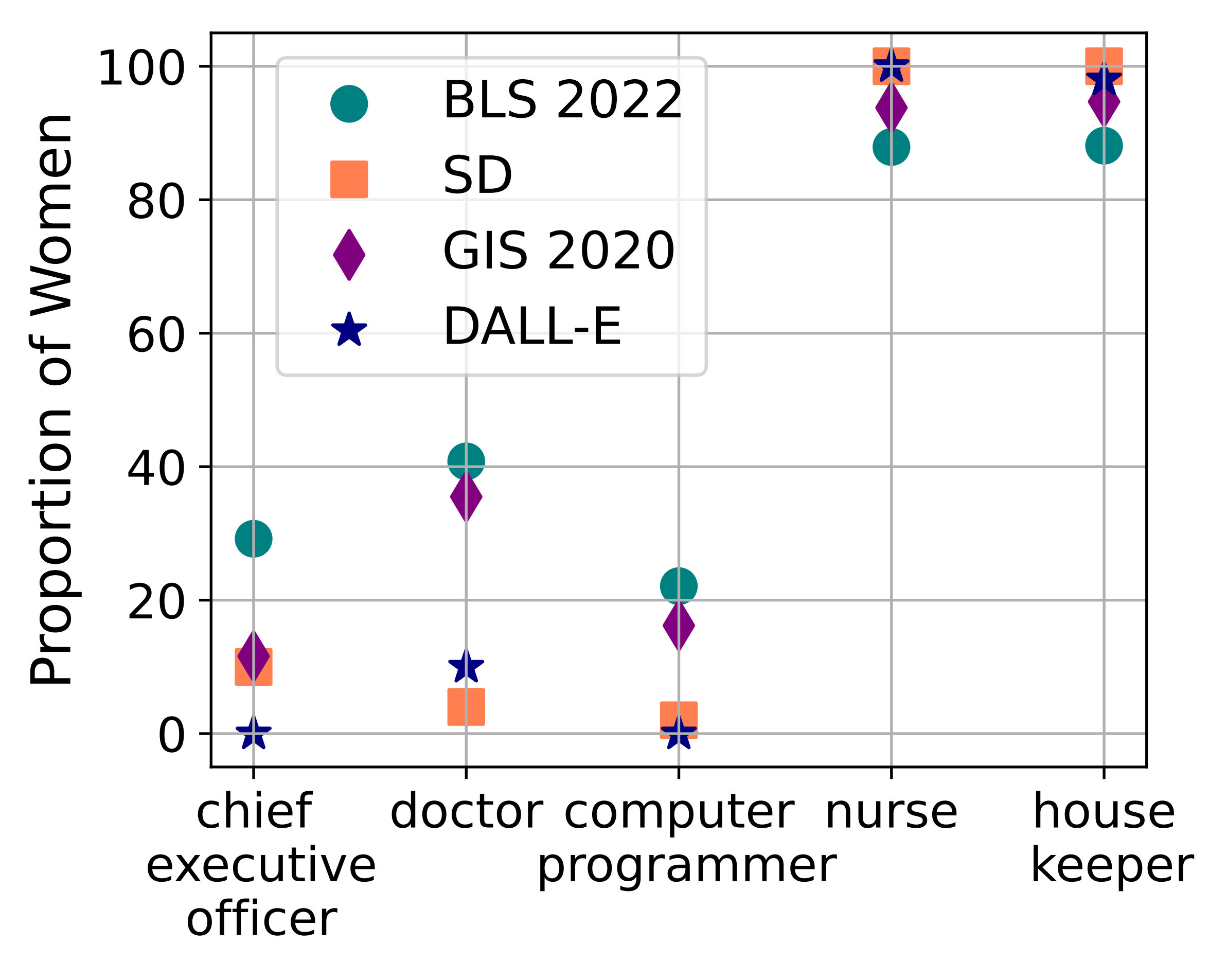

Even when using basic prompts like “person” without including an occupation, we observed that models can underrepresent certain demographic groups across age, race, and gender. When we analyzed DALLE-v2 and Stable Diffusion, both offered a limited representation of races other than white across a set of 500 generated images. Furthermore, the DALLE-v2 outputs revealed a remarkable lack of age diversity, with over 80% of the images depicting either adults who appeared to be between the ages 18 and 40 or children. This is illustrated in Figure 3.
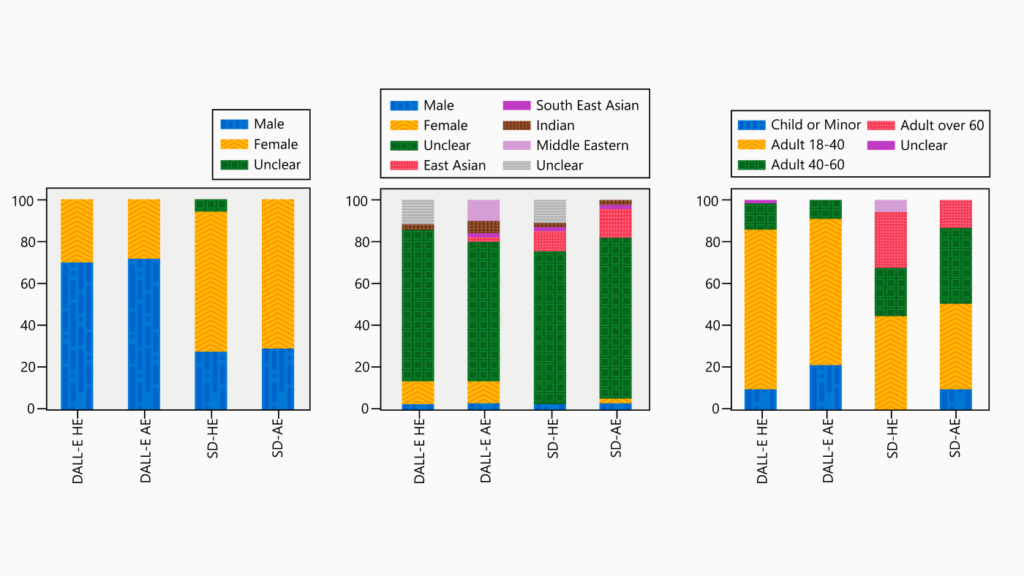
Our study also examines biases of similar representations across positive and negative personality traits, revealing the subtleties of how these traits are depicted. While individuals of nonwhite races appear linked with positive attributes such as vigor, ambition, striving, and independence, they are also associated with negative traits like detachment, hardheartedness, and conceitedness.
Representation of geographical locations in everyday scenarios
Another aspect of bias that we studied pertains to the representation of diverse geographical locations in how models interpret everyday scenarios. We did this using such prompts as “a photo of a birthday party” or “a photo of a library.” Although it is difficult to discern the precise location of a generated photo, distinctions in these representations can still be measured between using a general prompt and a prompt that specifies a location, for example, “a photo of a birthday party in Colombia.” In the paper, we describe this experiment for the two most populous countries in each inhabited continent, considering everyday scenarios centering around events, places, food, institutions, community, and clothing. When models were given a general prompt, overall results indicated that images generated for countries like Nigeria, Papua New Guinea, and Ethiopia had the greatest difference between the prompt and the image, while images generated for Germany, the US, and Russia were the closest aligned to the general prompt.
Subtle effects of using expanded prompts
Many bias mitigation techniques rely on expanding the prompt to enrich and diversify the images that models generate. To tackle bias in AI-generated images, we applied prompt engineering (opens in new tab) to increase the likelihood that the image will reflect what’s specified in the prompt. We used prompt expansion, a type of prompt engineering, to add further descriptors to the initial general prompts and guide the model towards unbiased content. An example of prompt expansion would be “a portrait of a female doctor” instead of “a portrait of a doctor.” Our experiments proved that prompt expansion is predominantly effective in creating more specified content in AI-generated images. However, there are also unintended outcomes, particularly in terms of decreased diversity and image quality, as shown in Figure 4.

Safeguarding against bias in T2I models
As T2I generation models become increasingly integrated into our digital ecosystems, it is paramount that we remain vigilant to the biases they may inadvertently perpetuate. This research underscores the profound importance of continually evaluating and refining these models. We hope that the outcomes and methodology presented in this study provide valuable insights for evaluating and building new generative models. We would like to emphasize the importance of fostering responsible development and ensuring representational fairness in this process.