Speech-to-speech translation promises to help connect our world
Among the futuristic gadgets in the classic TV show Star Trek, none seemed more useful than the universal translator, a handheld gizmo that helped foster understanding among intergalactic civilizations. Well, we needn’t travel beyond the solar system to find the need for such a device. Imagine being able to speak in English and have your thoughts expressed in grammatically and semantically correct Mandarin or Spanish. Then imagine the voice that expresses those translated thoughts is your own.
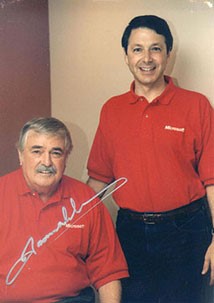
Long before unveiling Microsoft’s answer to the universal translator, the company’s chief research officer, Rick Rashid, was a Trekkie, delighted to pose in 1996 with one of Star Trek’s stars, the late James Doohan, who played Scotty.
Just another sci-fi fantasy? Maybe not. Thanks to work going on today at Microsoft Research, such speech-to-speech translation is moving ever closer to reality. One day in the not too distant future, you might ask about your dinner options in a Parisian restaurant, give detailed directions to a taxi driver in Moscow, or discuss a business deal with potential partners in Tokyo—fluently, in your own voice, without knowing a word of French, Russian, or Japanese. Your tablet or smartphone will do the heavy lifting of understanding what you’re saying in English, translating it into your listeners’ tongue, and speaking it in your voice with the pronunciation, tones, and inflections of a native speaker.
All this will be made possible by combining three key pieces of technology: speech recognition, language translation, and speech synthesis. Underlying it all are breakthroughs in machine learning, particularly the development of computer-based “deep neural networks.”
Start with speech recognition
Everything starts with the ability of your computing device to understand what you’re saying. Anyone over the age of 30 undoubtedly will recall the days of frustratingly imperfect speech recognition. Just to refresh your memory, though, here’s an actual log of a conversation between a human being and a computerized helper named “Annie”:
Computer: Annie here.
Human: Hello, Annie, could you give me information on a meeting I have tomorrow?
Computer: What was that? [The software failed to understand what had been said.]
Human: I need to find out, uh, a meeting time and place. Kim sent me a message on it.
Computer: For all options, say “Help me out.” [The software failed to understand what had been said.]
Human: Help me out.
Computer: Annie here. [The software failed to understand what had been said.]
Human: Help.
Computer: Help. Say “Call” or say “Send a message,” followed by a label or a number. Or say “Get my messages.”
Human: Get messages.
Computer: Speech and Image Processing Lab. [The software heard “Get me sips.”]
Human: Help.
Computer: Help. Say the first and last name …
So it went, back in the 1980s, when computerized systems stumbled while trying to understand the simplest of spoken requests. Such systems worked—sort of—in situations in which interactions between human and machine could be confined to a few repeated commands, which were often elicited by the machine, as in Say “Balance due,” “Payment date,” or “Recent transactions.” Conversely, the systems blew a linguistic fuse when a frustrated human replied with something like “I want to dispute a charge.” The error rate for recognizing speech that went outside the defined requests was unacceptably high.
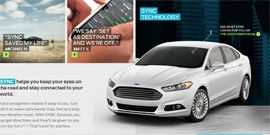
Improvements in speech recognition have brought voice-activated assistants to our smartphones, such as Ask Ziggy on Windows Phone, and voice commands to our cars, such as Ford SYNC.
By the mid-1990s, things had improved markedly. For example, Frank Seide, a multilingual computer scientist now a senior researcher at Microsoft Research Asia, participated in a project for an automated telephone system that provided train-schedule information to German callers. This system, and others like it, achieved acceptable results, albeit within a limited universe of spoken requests.
Today, things are better. You can speak a message into a smartphone and know that the software will convert it to text with an acceptable degree of accuracy. And we’ve all seen smartphone apps such as Apple’s Siri and Windows Phone’s Ask Ziggy, which act on our spoken requests with often astonishing accuracy. Granted, speech recognition is still not perfect—but then again, we frequently misunderstand what other people are saying to us, and our brains are a lot more sophisticated than the software in our smartphones.
Getting to today’s level of speech-recognition accuracy involved a major breakthrough in machine learning. Before 2006, developers trained speech-recognition systems by using techniques based on complex statistical constructs known as Gaussian mixture models (GMMs). Theoretically, this approach should lead to acceptable automatic speech recognition. In practice, though, the results have been frustrating.
All this began to change in 2006, with work conducted by Professor Geoffrey Hinton at the University of Toronto. He and his colleagues took a different approach to machine learning, using deep neural networks (DNNs), in which the computerized “brain” consists of many interconnected, hidden layers.
Li Deng, a principal researcher at Microsoft Research Redmond, had come to know Hinton while teaching at Canada’s University of Waterloo. Deng and Hinton continued their association after Deng joined Microsoft. In late 2009, Deng invited Hinton to Microsoft Research to work with him on the use of DNNs for speech recognition. This collaboration led to the discovery that while DNN models of speech recognition did not significantly lower error rates when compared to GMMs, they did result in a distinctly different pattern of errors—a pattern that interfered less with the reliability of the output. This discovery motivated Deng and Dong Yu, a senior researcher at Microsoft Research Redmond, to continue researching DNNs for speech recognition, and, during the summer of 2010, Yu, Deng, and George Dahl, a graduate student of Hinton’s, worked to extend the DNN models to large vocabularies, in order to tackle real-world scenarios of voice search. In the fall of that year, Seide and his colleagues from Microsoft Research Asia joined Yu in developing efficient, large-scale prototypes of DNN-based speech recognizers.
The first successes in large-scale, DNN-based recognizers were reported in 2010, with Yu, Deng, and Dahl publishing their research on context-dependent DNNs, involving networks with hundreds of output units, and 2011, when Seide, Microsoft Research Asia colleague Gang Li, and Yu reported on their work with a huge number of outputs and improved training models. The impact of these advances in speech recognition was drastic, reducing the word-error rate by a third when compared with the previous state of the art of GMMs. By 2013, DNN-based models had come close to halving the error rate compared with GMMs.
This new level of performance from the DNN-based speech recognizers, coupled with advances in the language-translation system such as that used in Bing Translator, motivated the Microsoft Research scientists to drive further progress in building a speech-to-speech translation system. Deng notes that DNNs crudely mimic the way our brains are constructed, with massive connections among various layers, as described in a 2012 paper in the IEEE Signal Processing Magazine:
The idea is to learn one layer of feature detectors at a time with the states of the feature detectors in one layer acting as the data for training the next layer. After this generative “pretraining,” the multiple layers of feature detectors can be used as a much better starting point for a discriminative “fine-tuning” phase during which backpropagation through the DNN slightly adjusts the weights found in pretraining.
While DNNs are good at processing certain data that human beings are also good at handling—particularly speech and vision—that doesn’t mean that DNNs learn in the same way as people do.
He conjures up the image of a multinational gathering, with everyone speaking in his or her own native tongue but being understood by everyone else.
Seide notes that “DNNs are ‘trained’ once and then kept constant, while humans keep learning throughout their life.” Both he and Deng are quick to add that the ability to create DNNs was the result of advances in the speed, memory, and processing power of computers.
While the move to DNNs produced a significant decline in the word-error rate of speech recognition, the approach is not perfect. Mistakes still happen. Not only have the number of errors dropped, the nature of the errors has changed—with far fewer mistakes of the sort that render the speech essentially nonsensical. The results are startlingly accurate when compared with the incoherence of previous approaches.
Hinton’s original work was largely theoretical, focusing on methodology. It was his collaboration with Microsoft Research that infused the work with a practical outlook, bringing what Deng calls “industrial scale” to the research. This shift to more practical applications has gone industrywide, with Microsoft competitors Apple, Google, and IBM all joining in the research and racing to “productize” improved speech recognition.
Bring in machine translation
Just as older attempts at automatic speech recognition left a lot to be desired, so, too, did past endeavors in automatic translation. As compelling as the promise of early translation software was, the results were almost always underwhelming.
Here are the opening lines of Gustave Flaubert’s classic French novel Madame Bovary, first in the original French, then as rendered by a professional translator, and finally as mangled by machine translation:
Original French
Nous étions à l’Étude, quand le Proviseur entra, suivi d’un nouveau habillé en bourgeois et d’un garçon de classe qui portait un grand pupitre. Ceux qui dormaient se réveillèrent, et chacun se leva comme surpris dans son travail.
English translation by human
We were in class when the headmaster came in, followed by a “new fellow,” not wearing the school uniform, and a school servant carrying a large desk. Those who had been asleep woke up, and every one rose as if just surprised at his work.
English translation by machine
We were in the study, when the headmaster came in, followed by a new dressed in bourgeois and a boy in class who wore a large desk. Those who slept awoke, and each stood as surprised in his work.
The machine translation shown here is based on current software and it obviously shows that there’s still ample room for improvement in machine-translation algorithms. Today, researchers are applying DNNs to the problem of translation, hoping to see the same kinds of improvements achieved in speech recognition. The outcome is uncertain, but with luck, it will soon spare us from hearing about a French student wearing a large desk.
Bing Translator for Klingon
In fact, machine translation has advanced to the stage where it’s a useful mobile app—to a point. It’s one thing to use your smartphone to ask for directions to the bathroom when you’ve had too much cerveza in Cabo. It’s quite another to expect it to help you negotiate a rental-car agreement in Spanish—let alone enabling you to converse on anything at length or in depth. That is exactly what Microsoft’s speech-to-speech project, which employs a state-of-the art translation engine built by Dongdong Zhang of Microsoft Research Asia, aspires to.
Add your own voice
So let’s see where we’re at with that universal translator. We’ve got the speech-recognition part in hand, thanks to the advances from using deep neural networks. And we’re hard at work on achieving acceptable machine translation. Can we make it speak in your voice?
Yes, we can, as Noelle Sophy and Henrique Malvar demonstrated in a small conference room at Microsoft Research’s Redmond headquarters. Sophy, a senior program manager, and Malvar, a Microsoft distinguished engineer and Microsoft Research’s chief scientist, described the progress that’s been made toward realizing effective speech-to-speech translation, particularly the work of Frank Soong, a principal researcher at Microsoft Research Asia. It’s Soong’s team, Malvar stresses, that has given speech-to-speech its “voice.”
Of course, seeing—or in this case, hearing—is worth a thousand words, so Sophy opens her laptop and plugs in a large microphone, the kind normally seen in recording studios. After waiting a few seconds for the software to load, she leans forward and speaks into the microphone, clearly and deliberately saying, “This is a demonstration of the Microsoft speech-to-speech system.” Her words appear on the laptop screen as soon as she utters them. Within seconds, Chinese characters also appear on the screen, and a voice speaks the translation of her words. The voice sounds uncannily natural, complete with the tonal inflections characteristic of Mandarin.
One problem, though—it doesn’t sound at all like Sophy’s voice. That’s because it isn’t. It’s actually the voice of Rick Rashid, Microsoft’s chief research officer and head of Microsoft Research. The reason why the software is using Rashid’s baritone instead of Sophy’s soprano is simple: The software has been trained on a sample of Rashid’s voice for use in a demo that’s become a YouTube sensation. Had the machine been trained against a sample of English speech from Sophy, the spoken Mandarin would have been in her voice.
Speech Recognition Breakthrough for the Spoken, Translated Word
Using a sample of Rashid speaking in English, the software—employing an approach developed by Soong—has broken his speech down into its elemental acoustics, which then can be recombined to make the sounds of spoken Mandarin. Next, the software does another neat bit of digital magic courtesy of Soong and his team, assembling Rashid’s sounds into Mandarin that rises and falls naturally. This natural-sounding cadence is the result of the words and sentences having been indexed against data acquired from a native Mandarin speaker. By mapping Rashid’s sounds to the natural patterns of spoken Mandarin, Soong’s speech-to-speech software has created an eerily accurate-sounding simulation of Rashid speaking Chinese. How accurate and natural sounding? The results have been tested with native Mandarin speakers, who confirm that the both the translation and the voice itself are amazingly natural. Hundreds of comments left on the YouTube video of Rashid’s demo further attest to the good quality of the translated speech.
The men behind the curtain
It was no accident that Rashid’s demo used translation from English to Mandarin. The speech-to-speech project began with work in Beijing, and the computer scientists at Microsoft Research Asia—particularly the two Franks, Seide and Soong—remain the driving force behind the project. Seide, a native German speaker who’s also fluent in English, and Soong, who’s bilingual in Mandarin and English, exude enthusiasm for their “baby.”
Seide talks excitedly about the potential of speech-to-speech translation, describing it as the fulfillment of “the dream of people talking to each other without language barriers.” He conjures the image of a multinational gathering, with everyone speaking in his or her own native tongue but being understood by everyone else. Soong adds an important caveat, noting that it’s vital for people to recognize that speech-to-speech currently is a research prototype, not a fully realized product.
How did this research effort get its start? It was fueled by the challenges of working in a cross-cultural, multilingual environment. As Seide describes, the project began with a system used to transcribe and then translate phone meetings between Microsoft researchers in Beijing and Redmond. Noting that the Chinese participants often were lost when they tried to follow the internal conversations taking place among the Redmond engineers, Seide and his colleague Kit Thambiratnam could see the value of a real-time spoken-translation application. They developed The Translating! Telephone, a precursor to the speech to-speech prototype.
The Translating Telephone, an early version of what would become the speech-to-speech translation demo.
Both Franks are quick to note that the Rashid demo simulates him speaking Mandarin. As Soong explains, “Rick doesn’t know Chinese, so no one can really say how he would sound if he spoke Mandarin.” Soong explains how the training sample used 2,000 sentences of Rashid speaking in English. Because there are a number of sounds in Mandarin that don’t occur in English, Rashid’s speech had to be “chopped up” into what Soong call tiles—acoustical snippets even smaller than phonemes, the basic phonological units that are combined into a language’s words. These tiles then were mapped against a reference speaker to give the illusion that Rashid was speaking Mandarin.
Needless to say, building a working prototype required a lot more than 2,000 sentences spoken by one person. This is, after all, a machine-learning process, and machine learning needs huge amounts of data to be effective. To train the system on English, the Beijing group licensed recordings of 2,000 hours of telephone calls, all made by paid callers and all painstakingly transcribed and paired with Mandarin translations. But even with this large body of data, the researchers confronted problems inherent in conversational speech, which differs markedly from written language. Conversation is full of starts and stops—uhs and ums and sentence fragments.
Related publications
- Roles of Pre-Training and Fine-Tuning in Context-Dependent DBN-HMMs for Real-World Speech Recognition
- Conversational Speech Transcription Using Context-Dependent Deep Neural Networks
- Context-Dependent Pre-trained Deep Neural Networks for Large Vocabulary Speech Recognition
- Deep Neural Networks for Acoustic Modeling in Speech Recognition
It’s a tricky proposition to design a real-time, automatic translation system that can handle the informality and grammatical lapses that characterize our everyday chats. So even the highly regarded demo involved Rashid speaking in what Seide calls “lecture style,” a formal mode of expression that ensured his speech would be grammatical. You also can notice that Rashid speaks one sentence at a time and then pauses, a technique that gives the software time to process his words and provide a reasonable translation. But this is a demo, after all, and it certainly shows the potential of this technology.
It’s hard to say when that potential will be realized. As Seide and Soong stress, the work is on an evolutionary path, one that already has gone a long way toward achieving speech-to-speech translation. While stressing that their work is pure research—intended to show the prospects of a speech-to-speech system—Seide and Soong recognize the enormous product potential. Imagine, muses Soong, the value of embedding this technology into a device for international travelers—it could, he says, “be useful in any number of everyday scenarios … and invaluable in emergency situations.” Seide adds that speech-to-speech translation could be extremely valuable during international conferences, especially smaller, specialized gatherings that don’t have the budget to hire human translators. He also offers a more personal potential use: It could facilitate communication with his Chinese in-laws, who speak neither English nor German.
Getting directions in a foreign city, hearing a foreign lecture in your own language, or enjoying a foreign film without subtitles: Speech-to-speech translation suggests all these possibilities, not to mention the potential to break down cultural and political barriers, which brings us back to Star Trek and the universal translator. If such a device can resolve extraterrestrial misunderstandings, surely it could do a world of good right here on Earth.
Adding more languages
Speech-to-speech translation is a prototype that works with one language pair: English and Mandarin. The goal is to bring in many more language pairs. Obvious candidates are widely spoken, commercially important tongues such as Spanish, German, or Japanese. But does that leave speakers of less prominent languages out in the cold? Not necessarily. Thanks to Microsoft Translator Hub, speakers of any language can build translation models that someday could find their way into speech-to-speech applications.