
Generative AI has revolutionized how we create text and images. How about designing novel materials? We at Microsoft Research AI4Science are thrilled to announce MatterGen, our generative model that enables broad property-guided materials design.
The central challenge in materials science is to discover materials with desired properties, e.g., high Li-ion conductivity for battery materials. Traditionally, this has been done by first finding novel materials and then filtering down based on the application. This is like trying to create the image of a cat by first generating a million different images and then searching for the one with a cat. In MatterGen, we directly generate novel materials with desired properties, similar to how DALL·E 3 tackles image generation.
MatterGen is a diffusion model specifically designed for generating novel, stable materials. MatterGen also has adapter modules that can be fine-tuned to generate materials given a broad range of constraints, including chemistry, symmetry, and properties. MatterGen generates 2.9 times more stable (≤ 0.1 eV/atom of our training + test data convex hull), novel, unique structures than a SOTA model (CDVAE). It also generates structures 17.5 times closer to energy local minimum. MatterGen can directly generate materials satisfying desired magnetic, electronic, mechanical properties via classifier-free guidance. We verify generated materials with DFT-based workflows.
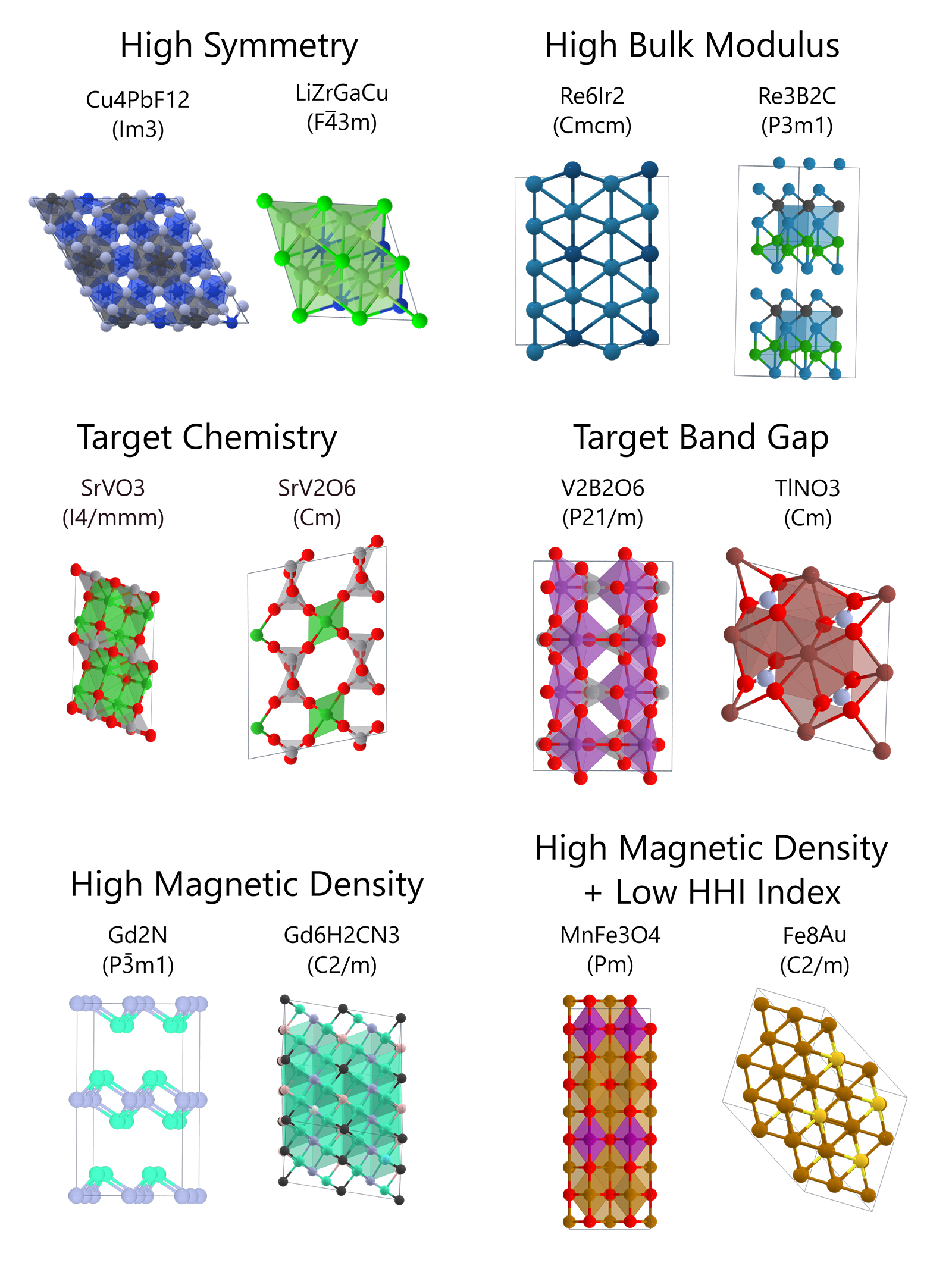
Microsoft research podcast
Collaborators: Silica in space with Richard Black and Dexter Greene
College freshman Dexter Greene and Microsoft research manager Richard Black discuss how technology that stores data in glass is supporting students as they expand earlier efforts to communicate what it means to be human to extraterrestrials.
Additionally, MatterGen can keep generating novel materials that satisfy a target property like high bulk modulus while screening methods instead saturate due to the exhaustion of materials in the database.
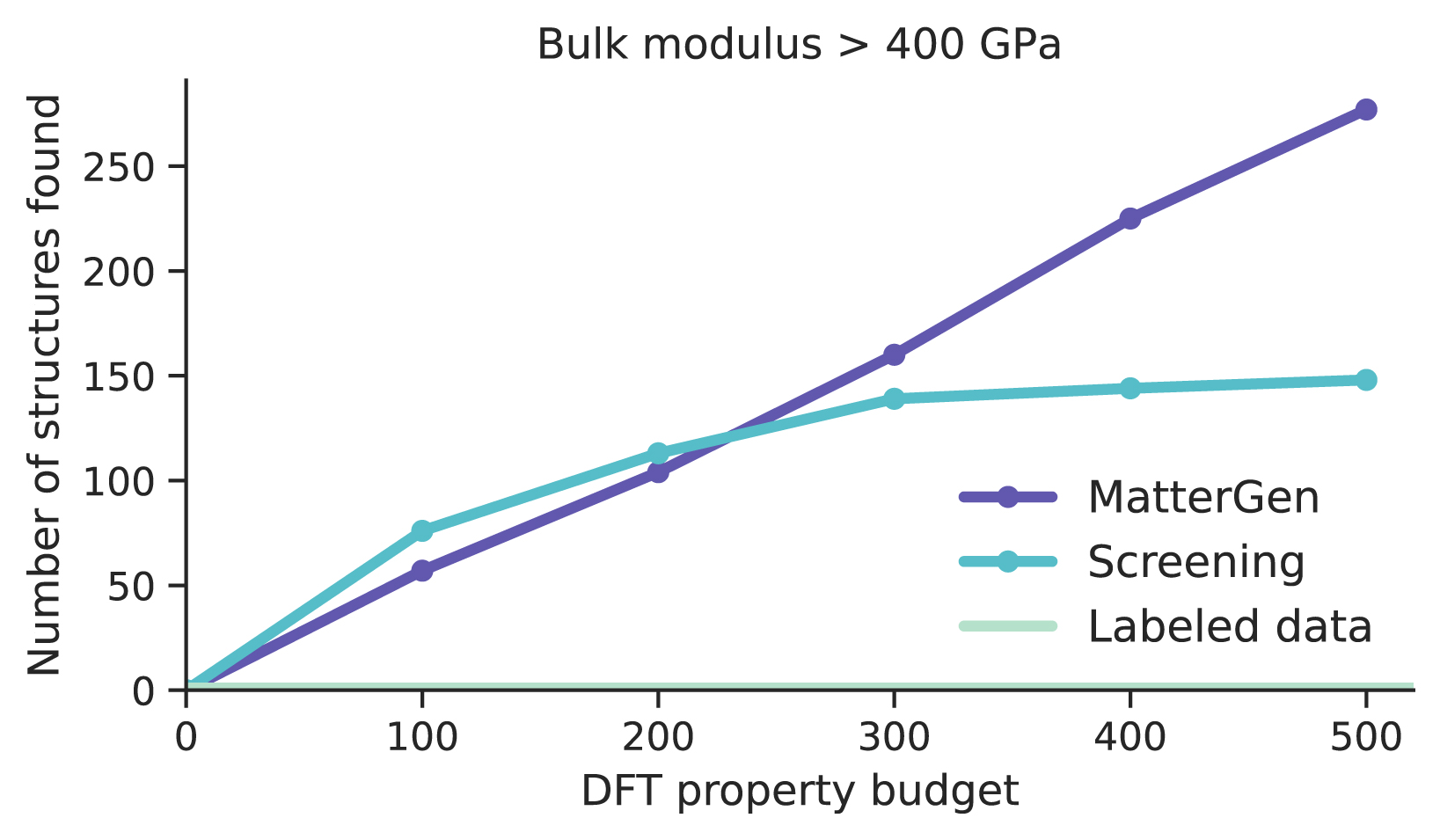
MatterGen can also generate materials given target chemical systems. It outperforms substitution and random structure search baselines equipped with MLFF filtering, especially in challenging 5-element systems. MatterGen also generates structures given target space groups. Finally, we tackle the multi-property materials design problem of finding low-supply-chain risk magnets. MatterGen proposes structures that have both high magnetic density and a low supply-chain risk chemical composition.
We believe MatterGen is an important step forward in AI for materials design. Our results are currently verified via DFT, which has many known limitations. Experimental verification remains the ultimate test for real-word impact, and we hope to follow up with more results.
None of this would be possible without the highly collaborative work between Andrew Fowler, Claudio Zeni, Daniel Zügner, Matthew Horton, Robert Pinsler, Ryota Tomioka, Tian Xie and our amazing interns Xiang Fu, Sasha Shysheya, and Jonathan Crabbé, as well as Jake Smith, Lixin Sun and the entire AI4Science Materials Design team.
We are also grateful for all the help from Microsoft Research, AI4Science, and Azure Quantum.