“Scottish independence: polls show it’s too close to call.”
“Scotland’s vote likely to be a nail-biter.”
“Scottish independence vote on a knife edge as polls put both Yes AND No ahead.”
If there was any consensus in the days running up to the momentous Sept. 18 vote in Scotland, it was that no one could predict the outcome. Headlines from Edinburgh, London, and across the globe were in complete agreement: It was impossible to say with any confidence what would happen.
And then there was David Rothschild (opens in new tab), a Microsoft researcher and leading expert in a new kind of data-driven predictive methodology. Three days ahead of the vote in Scotland, he put the chances of a No outcome at 77.4 percent. Two days later, he inched it up to 79.5. On the morning of the vote, before any returns were announced, he went on record on his blog (opens in new tab) with an 84 percent chance of defeat for Scottish independence.
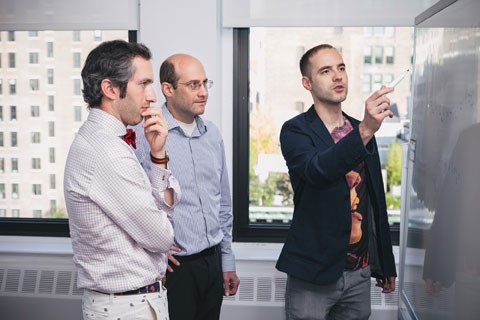
Miro Dudik (at whiteboard) confers with Microsoft Prediction Lab colleagues David Rothschild (left) and David Pennock.
This isn’t a mere parlor game for Rothschild, who, along with colleagues at Microsoft and elsewhere, correctly predicted the winners of all 15 World Cup knockout games earlier this year and got the Obama vs. Romney outcome right in 50 of 51 jurisdictions (the states plus the District of Columbia) in the 2012 U.S. presidential election. It seems no contest is beyond the purview of Rothschild’s predictive powers, whether it’s congressional races, the Super Bowl, the Oscars, or the Eurovision Song Contest.
In an era in which traditional political polling is taking a huge reputational hit—just ask Eric Cantor, former majority leader of the U.S. House of Representatives, who lost his Republican primary election in Virginia by 11 percentage points despite his own pollster putting him 34 points ahead—Rothschild’s success rate is gaining notice.
That momentum culminates this week with the launch of a new, interactive platform, Microsoft Prediction Lab, which serves as a website-based showcase (opens in new tab) and as a laboratory for his ongoing work.
“The polls track the sentiment of the people who are answering the poll at the time,” Rothschild said as he awaited the results in Scotland. “My forecast predicts what will happen on Election Day. Clearly, the sentiment of the people at the time of the polls is a critical component on any forecast of Election Day, but not the only one.”
“It may actually be reasonably convincing,” he said of the victory for the No side. And convincing it was: 55 to 45 percent.
The Problem with Representational Polling
Consider conventional political polling, which has a solid track record but is expensive and time-consuming. In recent decades, polling companies have relied on random-digit landline phone calls to determine voter sentiment. The accuracy of such results depends significantly on reaching a representative sample of people who actually will go to the polls. In the era of mobile phones and caller ID, the obstacles are mounting.
Among the insights that Rothschild has documented and that he puts to considerable use in his methodology is that polls of voters’ expectations—who they think will win—is a more accurate basis for forecasting than polls asking people how they intend to vote.
“[T]his is because we are polling from a broader information set, and voters respond as if they had polled 20 of their friends,” he wrote in a 2013 paper (opens in new tab) co-written with Justin Wolfers of the University of Michigan. Not surprisingly, then, Rothschild regularly includes data from betting markets in generating his predictions, including his forecast of the Scottish independence vote.
Another major contribution from Rothschild, who has a doctorate in applied economics from the Wharton School of Business at the University of Pennsylvania, is that by applying the appropriate statistical adjustments, highly unrepresentative samples can be used to generate remarkably accurate forecasts.
He and several colleagues demonstrated this in a novel experiment (opens in new tab) that polled Xbox (opens in new tab) users before the 2012 U.S. presidential election. They conducted an opt-in poll in the 45 days before the election and enabled people to participate once a day. In addition to asking, “If the election were held today, who would you vote for?” they collected basic demographic information: sex, race, age, education, state of residence, party identification, political leanings, and how the respondent voted in the 2008 presidential election.
As you might expect, the vast majority of Xbox users—and thus survey respondents—were male and relatively young. They would make a terrible sample for standard polling. But they served the researchers’ purposes.
“Standard polling looks at a respondent as, for example, a male from New York,” Rothschild says. “The way we look we look at it is: a male and a person from New York. I hope to find other potential polltakers who are male and other potential polltakers who are from New York. And from that, by breaking people into their demographics, we’re able to allow all users to inform the likely polling of all other users.”
So even though they were short on women older than 65, for example, they had a number of female respondents and some respondents older than 65, along with others who shared certain other characteristics with older women. In the end, the data from more than 750,000 Xbox surveys taken by almost 350,000 unique respondents yielded 176,000 different demographic “cells,” each with a distinct combination of characteristics.
From there, the researchers “post-stratified” the Xbox responses to mimic a representative sample of likely voters, calculating cell weights by cross-tabulating with exit polls from the 2008 presidential election. As Election Day approached, they used the accumulated data to update their forecasts daily for each state.
“Not only did we match the accuracy of major polling companies,” Rothschild says, “but we also provided a lot of insight that they weren’t able to get, through the fact that we had people coming back again and again.”
Each predictive exercise that Rothschild runs draws from a different pool of data, which is often a combination of polling data, historical results, Internet betting data, routinely collected statistics, and user-generated data. For Major League Baseball playoffs, for example, massive amounts of data are available from the regular season. World Cup soccer doesn’t have that kind of buildup, so it makes sense to engage the crowd to collect new data to augment historical data about the players and teams and the results of the qualifying rounds.
“There’s always something missing—always data we wish we had that didn’t quite exist,” Rothschild says. “So we’ve done a lot of fun experiments.” These include Oscars prediction games and NFL prediction games that were designed to attract people with a high level of expertise in those areas.
“The way I’ve always looked at it,” Rothschild says, “is that any individual—you, me, the guy on the street—has a certain amount of information about the things the person cares about, but no one has been unlocking it.”
The conventional pollsters “don’t think about somebody who is self-selected,” he explains. “They go to random people. They also use very simple aggregation methods, rather than modeling the results they have. That’s what computers are for. That’s what our new knowledge is for.”
Rothschild and his colleagues apply deep expertise in machine learning to test and calibrate their models against historical data, and they use advanced algorithms to account for a host of variables, such as the advantages of incumbency and the tendency of bettors to overstate long-shot wins.
Reinventing Survey Research
The interactive platform that Rothschild and other researchers launched today houses all of the ongoing predictive work that Rothschild has been featuring on his blog and in academic journals and presentations. The Microsoft Prediction Lab displays his data-driven predictions—some of them updated in real time—in a wide range of fields, from sports and entertainment to politics and economics.
“We’re building an infrastructure,” he says, “that’s incredibly scalable, so we can be answering questions along a massive continuum.”
Rothschild sees the new platform as “a great laboratory for researchers” as well as “a very socialized experience” for interested users. Among other contests, he plans to predict the results of every upcoming U.S. House, Senate, and gubernatorial race. Users will be able to customize views on the site based on their geographic location and their interests. The idea is to collect data quickly and update it as often as possible.
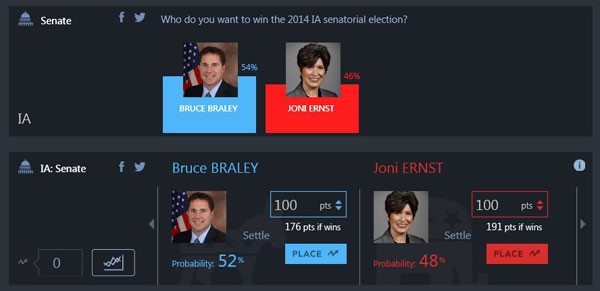
A sample of the Microsoft Prediction Lab interface users will see for every U.S. House, Senate, and gubernatorial race in 2014.
“It’s also important to be agnostic and not be wed to one type of data,” Rothschild says. He looks at any data that can contribute to the predictive model, whether it’s stock-market data, Internet page views, or trending topics and word co-occurrence on social media. Collecting “crowd wisdom” will be a big component of the endeavor.
“By really reinventing survey research, we feel that we can open it up to a whole new realm of questions that, previously, people used to say you can only use a model for,” Rothschild says. “From whom you survey to the questions you ask to the aggregation method that you utilize to the incentive structure, we see places to innovate. We’re trying to be extremely disruptive.”
That disruption has ramifications for the polling industry—and beyond.
“There are two reasons to experiment with nonprobability polling,” he says. “First, I firmly believe the standard polling will reach a point where the response rate and the coverage is so low that something bad will happen. Then, the standard polling technology will be completely destroyed, so it is prudent to invest in alternative methods.
“Second, even if nothing ever happened to standard polling, nonprobability polling data will unlock market intelligence for us that no standard polling could ever provide. Ultimately, we will be able to gather data so quickly that the idea of a decision-maker waiting a few weeks for a poll will seem crazy.”
The ready availability of such data will enable businesses to make strategic investment decisions, such as where to locate a data center or how to invest marketing resources to attain the optimal yield.
“We will be able,” Rothschild says, “to gather so much detail from repeated users—and the quantity of users we can reach—that decision-makers will come to cherish the nearly infinite number of data points that can be efficiently generated to answer the exact questions the question-maker has, not the expedient question or the historical norm.”
One caveat, though: The market intelligence derived from the nonprobability polling data must prove accurate.
“That is what this research is all about,” he adds, “reaching that point where the quick, relevant, and cost-effective market intelligence is as accurate as what it supplants. At that point, the demise of standard polling becomes irrelevant, because it will become strictly dominated by nonprobability data collection and analytical techniques.”
The new Microsoft Prediction Lab website draws on the expertise of researchers in Microsoft’s New York City (opens in new tab), Redmond (opens in new tab), and India (opens in new tab) labs. Key contributors include noted computer scientists Miro Dudík (opens in new tab) and David Pennock (opens in new tab), as well as a research team led by Harry Shum (opens in new tab), Microsoft executive vice president of Technology and Research, and the office of Microsoft’s chief economist, Preston McAfee (opens in new tab).
“It has been,” Rothschild confirms, “an incredibly collaborative effort.”
“Most researchers get the opportunity to explore a much more narrow set of questions and a much more narrow set of data,” he says. “But through collaboration with an awesome set of researchers, this really allows me to explore things that are so buried. And that’s really the most exciting thing about this. It’s not any individual outcome—it’s the massive amount of questions that we’ll be able to answer in the near future.”