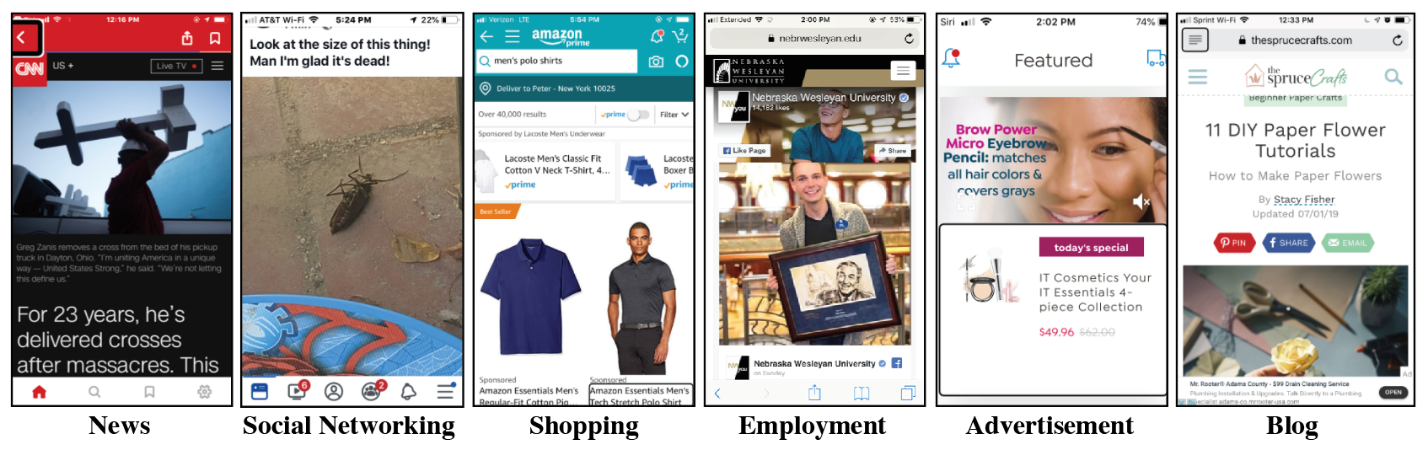
In a study about the kinds of details people who are blind or have low vision want when encountering digital images, researchers used several different types of sources as prompts. The above are examples of images participants encountered when browsing some of those sources. Participants reported desiring image descriptions that varied in detail depending on the context in which an image appeared.
Image descriptions are vital to making digital content fully accessible to people who are blind or have low vision. However, as past research has shown, content authors often leave out these descriptions—also known as “alternative text” or “alt text”—for images on the web (opens in new tab) and on social media (opens in new tab), making AI-based vision-to-language services that automatically generate image descriptions particularly important. To learn more about the design requirements for AI systems to create useful descriptions, our research team conducted interviews with 28 people who are blind or have low vision about the types of details they want when encountering digital images and identified ways in which those wants vary depending on where an image is encountered.
Our findings are presented in the paper “’Person, Shoes, Tree. Is the Person Naked?’ What People with Vision Impairments Want in Image Descriptions,» (opens in new tab) which was accepted at the ACM CHI Conference on Human Factors in Computing Systems (CHI 2020) (opens in new tab). The work is part of the Microsoft Ability Initiative (opens in new tab), a two-year collaboration launched last year between Microsoft researchers in accessibility and computer vision and faculty and students in AI and human-computer interaction at The University of Texas at Austin. Funded by Microsoft Research and AI for Accessibility Program (opens in new tab), the initiative aims to increase the utility and usability of automatically generated image descriptions for people who are blind, a population that relies on screen reader technology to access computing devices. Screen readers present textual content as audio or Braille output and can only present digital images if they have an accompanying alt text description.
The paper’s title stems from one participant’s observation regarding the inadequacy of current AI-based image descriptions. In the tag-based approach used by Facebook, for example, images are labeled with statements such as “This image may contain: a person, shoes, a tree.” In describing their encounters with such labels, the participant wondered in a tongue-in-cheek way whether the person in the image was really wearing only shoes, highlighting the lack of critical details in today’s AI-generated descriptions.
“In this work, our first priority was to learn from people who are blind or have low vision about their daily experiences with image descriptions,” said our lead author on the paper, postdoctoral researcher Abigale Stangl from UT Austin (opens in new tab). “The AI for Accessibility program enabled us to take the time to hear from teachers, lawyers, music producers, students, politicians, job seekers, and others and then communicate our empirical findings in a way that can improve computer vision algorithms.”
Same image, different details
While earlier studies have identified that the usefulness of image descriptions is dependent on the digital context in which the image appears, our study gives insight into how image description wants vary based on context across seven different sources: news websites, social networking sites/platforms, e-commerce websites, employer/employment websites, online dating websites/platforms, productivity applications, and e-publications.
“Though it is well understood that image descriptions are important to convey the purpose of an image, this research showed us that people who are blind or have low vision want image descriptions that are responsive to where they encounter the image,” said Stangl. “In other words, people want different content for the same image depending on where they find it.”
For example, if a photo of a person appeared in a news story, people might want a description that includes details about the setting of the image to give a sense of place. But if a photo of a person appeared on a social media or dating website, people might want increased details about that person’s appearance, including some details that may be subjective and/or sensitive, such as race, perceived gender, and attractiveness. One participant mentioned that knowing the race and gender of people in photos of board members on an employer/employment website might help them understand whether the company values a diverse workplace. These latter examples illustrate practical and ethical challenges for emerging AI systems, such as whether AI systems can—or should—be trained to provide subjective judgments or information about sensitive demographic attributes.
News | Social Networking | eCommerce | Employment | Dating | Productivity | E-Publication | |
Event/Scene | |||||||
People Present | x | x | x | x | x | x | x |
Text | x | x | x | x | x | x | x |
Activity | x | x | x | x | x | x | |
Interaction | x | x | x | x | x | ||
Landmarks | x | x | x | x | x | ||
Building Features | x | x | x | x | x | ||
Weather | x | x | x | x | |||
Lighting | x | ||||||
People | |||||||
Text | x | x | x | x | x | x | x |
Salient Objects | x | x | x | x | x | x | |
Activity | x | x | x | x | x | x | |
Gender | x | x | x | x | x | ||
Race/Diversity | x | x | x | x | x | ||
Name of Person | x | x | x | x | |||
Celebrity Name | x | x | x | x | |||
Expression | x | x | x | x | |||
Attire/Clean | x | x | x | ||||
Body Shape/Size | x | x | |||||
Pets | x | x | |||||
Hair Color | x | ||||||
Hair Style | x | ||||||
Eye Color | x | ||||||
Unique Physical | x | ||||||
Tattoos | x | ||||||
Object | |||||||
Text | x | x | x | x | x | x | x |
Name | x | x | x | x | x | x | x |
Form | x | x | x | ||||
Fit | x | x | x | ||||
Color | x | x | x | ||||
Overall Style | x | x | x | ||||
Material | x | x | x | ||||
Logos/Symbols | x | x | |||||
Damage | x | ||||||
Unique Features | x |
The above table is a cross-source analysis of participants’ description preferences, indicated with an x, for seven source types. The preferences are grouped by common focuses of image composition—event/scene, people, and objects—and the types of preferences were included if at least one participant indicated them as important to include.
As part of our research, we created a tabular reference guide identifying the types of details people find useful across sources. These findings can inform the design of future AI-based captioning tools. For instance, the details of interest to our participants suggest new categories of metadata that should be produced by crowd workers to feed into improved machine learning models. Our work also highlights the opportunity for creating custom vision-to-language models that are dependent on the context in which an image appears.
Accelerating advancement
The Microsoft Ability Initiative with UT Austin is part of the Microsoft Research Ability team’s (opens in new tab) ongoing focus on image accessibility (opens in new tab). In addition to this research, we’re jointly hosting an Image Captioning Data Challenge (opens in new tab) and VizWiz Grand Challenge Workshop (opens in new tab) as part of the International Conference on Computer Vision and Pattern Recognition (CVPR 2020) (opens in new tab), which is scheduled to take place in Seattle in June.
“We are excited that this event will promote greater interaction between the diverse groups of researchers and practitioners working on image description technologies,” said co-author and UT Austin professor Danna Gurari (opens in new tab). “Ultimately, we expect this work will accelerate the conversion of cutting-edge research into market products that better empower people who are blind or have low vision to independently address their daily visual challenges.”