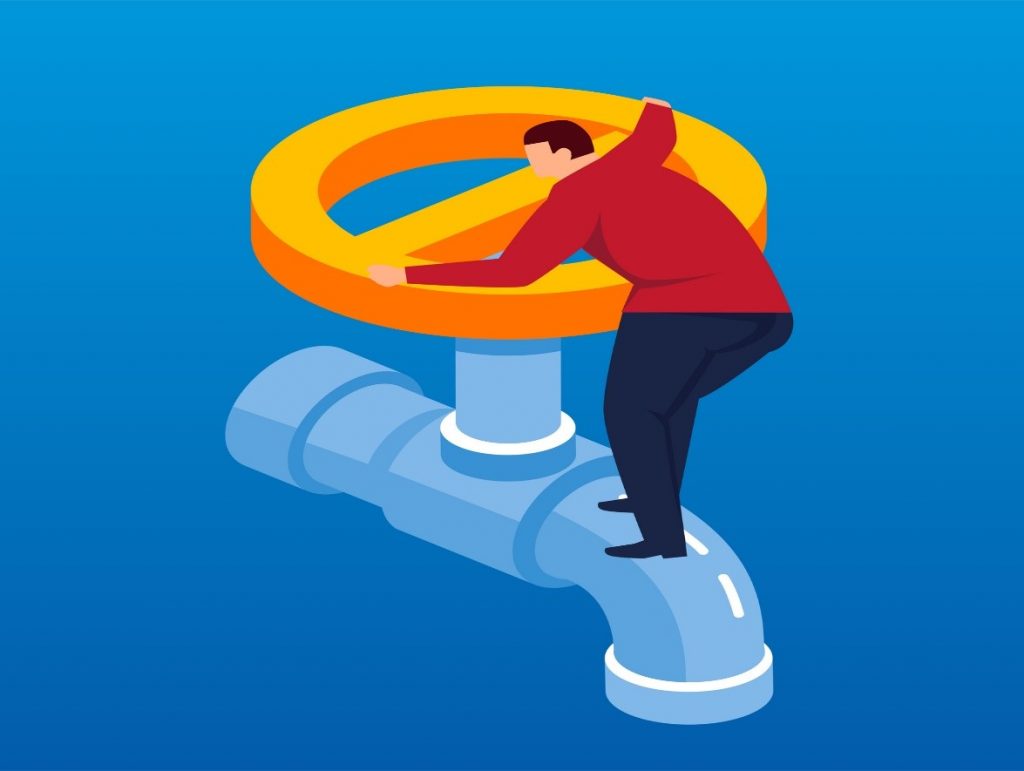
The fraud detection paradox—to tighten or loosen
As mentioned in my Banking Use Case: Detecting Online and Mobile Fraud with AI, banks can approach the problem of false positives by 1) drastically lowering fraud detection thresholds, which can increase the rate of false positives, or 2) tightening fraud detection models to lower the number of false positives, which increases the likelihood of missing real fraud. Hence, the fraud paradox.
Regulators are cracking down harder on banks through increased enforcement actions. But missed transactions can be catastrophic to a bank’s brand and bottom line. Financial institutions must find new ways to implement online and mobile fraud detection capabilities across all customer databases, payment channels, and transactional systems.
To more aggressively stop criminal transactions, banks are shifting from a passive methodology of “observe, react, and report” to a proactive approach of blocking fraud before it occurs. However, this strategy risks producing higher rates of false positives, and the system might mistakenly freeze a customer’s legitimate transaction. Every time a legal transaction is blocked, the bank induces a negative impact on customer satisfaction—and that increases the probability of customer churn.
The challenge is to maintain a balance of meticulous and rapid detection without interrupting legitimate customer transactions. Banks face enormous pressures to satisfy the customer experience with fraud detection. The new goal is precise, real-time detection. But getting to detection nirvana—100% fraud detection—requires management tools that are easy to use. The trend is toward graphical dashboards showing risk levels, and seamless integration with multiple business systems. The real goal is accessible insight. Not only do we want to find the needles in the haystack, but we want them laid out before us. A bonus would be to see the logic behind the selection, so the user can quickly decide to unblock a transaction or take other actions.
Another approach is to use sampling for fraud detection, but it has a drawback. The flaw is that fraudulent transactions do not occur randomly. So, a bank could find one transaction to be fraudulent, but miss three more that were in sequential order. Therefore, sample detection could miss some fraudulent transactions. In addition, sampling does not fully account for multiple occurrences of fraud within the current populations of transactions—hindering probability models or estimates. Banks could also omit several much smaller, less frequent fraudulent transactions adding up to be extremely large fraud losses in aggregate.
The business of fraud detection is not easy. From the evolving forms of fraud to the growing complexity of illegal transactions, banks are caught in the land of “no easy answers.” There is no right or wrong answer to how to throttle fraud detection. Every bank has different risk tolerances and variables beyond that one customer transaction. However, leveraging cloud capabilities increases the capacity to handle higher volumes of transactions, and everchanging fraud tactics. Systems built on Azure are the first choice for better management of the fraud detection paradox.
Want to know about other areas of financial crime, such as online and mobile fraud and how cloud capabilities can help? Read Banking Use Case: Detecting Online and Mobile Fraud with AI, which provides actionable recommendations and solutions, and then engage with the author on this topic by reaching out to me on Twitter and LinkedIn.